Accepted Manuscript
Title: Brain alterations in children/adolescents with ADHDrevisited: a neuroimaging meta-analysis of 96 structural andfunctional studies
Authors: Fateme Samea, Solmaz Soluki, Vahid Nejati,Mojtaba Zarei, Samuele Cortese, Simon B. Eickhoff, MasoudTahmasian, Claudia R. Eickhoff
PII: S0149-7634(18)30662-6DOI: https://doi.org/10.1016/j.neubiorev.2019.02.011Reference: NBR 3351
To appear in:
Received date: 31 August 2018Revised date: 21 January 2019Accepted date: 16 February 2019
Please cite this article as: Samea F, Soluki S, Nejati V, Zarei M, Cortese S,Eickhoff SB, Tahmasian M, Eickhoff CR, Brain alterations in children/adolescentswith ADHD revisited: a neuroimaging meta-analysis of 96 structuraland functional studies, Neuroscience and Biobehavioral Reviews (2019),https://doi.org/10.1016/j.neubiorev.2019.02.011
This is a PDF file of an unedited manuscript that has been accepted for publication.As a service to our customers we are providing this early version of the manuscript.The manuscript will undergo copyediting, typesetting, and review of the resulting proofbefore it is published in its final form. Please note that during the production processerrors may be discovered which could affect the content, and all legal disclaimers thatapply to the journal pertain.
1
Brain alterations in children/adolescents with ADHD revisited: a neuroimaging meta-
analysis of 96 structural and functional studies
Fateme Samea1 M.Sc., Solmaz Soluki1 M.Sc., Vahid Nejati1,2≠ Ph.D., Mojtaba Zarei3 MD., Ph.D.,
Samuele Cortese4,5,6,7 M.D., Ph.D., Simon B. Eickhoff8,9 M.D., Masoud Tahmasian3*≠ M.D., Ph.D.,
Claudia R. Eickhoff9,10,11 M.D.
1 Institute for Cognitive and Brain Sciences, Shahid Beheshti University, Tehran, Iran.
2 Department of Psychology, Shahid Beheshti University, Tehran, Iran.
3 Institute of Medical Science and Technology, Shahid Beheshti University, Tehran, Iran.
4 Center for Innovation in Mental Health, Academic Unit of Psychology, University of Southampton,
Southampton, UK.
5 Faculty of Medicine, Clinical and Experimental Sciences (CNS and Psychiatry), University of
Southampton, Southampton, UK.
6 Division of Psychiatry and Applied Psychology, School of Medicine, University of Nottingham,
Nottingham, UK.
7 Department of Child and Adolescent Psychiatry, NYU Langone Medical Center, New York, USA.
8 Institute for Systems Neuroscience, Medical Faculty, Heinrich-Heine University Düsseldorf, Germany.
9 Institute of Neuroscience and Medicine (INM-1; INM-7), Research Center Jülich, Jülich, Germany.
10 Institute of Clinical Neuroscience and Medical Psychology, Heinrich Heine University Düsseldorf,
Düsseldorf, Germany.
11 Department of Psychiatry, Psychotherapy, and Psychosomatics, RWTH Aachen University, Aachen,
Germany.
≠ V.N. & M.T. contributed equally to this study.
* Corresponding author: Masoud Tahmasian M.D., Ph.D., Institute of Medical Science and
Technology, Shahid Beheshti University, Daneshjou Boulevard, Velenjak, P.O. Box
1983969411, Tehran, Iran. Telephone: +98-21-29905803; Fax: +98-21-29902650;
Email: m_tahmasian@sbu.ac.ir
Running title: Brain alterations in children/adolescents with ADHD
Count: Title (16), Abstract (168), Text (4787), References (49), Figure (3), Table (1),
Supplement file (1).
Highlights
ACCEPTED MANUSCRIP
T
2
The results of neuroimaging studies in children/adolescents with Attention-
Deficit/Hyperactivity Disorder (ADHD), and those of meta-analyses provide mixed
conclusions. Here, we conducted the largest meta-analysis including both
structural and functional MRI, using state of the art procedures and following
recently established consensus guidelines for neuroimaging meta-analyses, to
address this inconsistency.
We found no significant convergent structural or functional alterations in ADHD in
the main analysis. Among several different sub-analyses, we only observed the
convergence dysfunction for task-fMRI experiments (using neutral stimuli) in the
left pallidum/putamen and decreased activity (using male subjects) in the left
inferior frontal gyrus.
In general, our results suggest either a real lack of consistent brain alterations
across patients or a more distributed, network-based pathology lacking a
consistent expression at any particular location, which might be due to
heterogeneous clinical populations, in-/exclusion criteria, various experimental
design, preprocessing, statistical procedures in individual publications.
This study highlights the need for further exploration in homogenous clinical
samples assessing regional structural and functional alterations, as well as
connectivity in parallel to unravel the relation between abnormal regional effects
and disturbed integration in children/adolescents with ADHD.
Abstract The findings of neuroimaging studies in children/adolescents with ADHD, and even those of previous
meta-analyses, are divergent. Here, Activation Likelihood Estimation meta-analysis, following the current
best-practice guidelines, was conducted. We searched multiple databases and traced the references up to
June 2018. Then, we extracted the reported coordinates reflecting group comparison between ADHD and
healthy subjects from 96 eligible studies, containing 1914 unique participants. The analysis of pooled
ACCEPTED MANUSCRIP
T
3
structural and functional, sub-analyses restricted to modality, and in-/decreased contrast did not yield any
significant findings. However, further sub-analyses in the task-fMRI experiments (neutral stimuli only) led
to aberrant activity in the left pallidum/putamen and decreased activity (male subjects only) in the left
inferior frontal gyrus. The overall findings indicate a lack of regional convergence in children/adolescents
with ADHD, which might be due to heterogeneous clinical populations, various experimental design,
preprocessing, statistical procedures in individual publications. Our results highlight the need for further
high-powered investigations, but may also indicate ADHD pathophysiology might rest in network
interactions rather than just regional abnormality.
Key words: ADHD; Activation likelihood estimation; Coordinate-based meta-analysis; fMRI;
VBM.
ACCEPTED MANUSCRIP
T
4
1. Introduction
Attention-deficit/hyperactivity disorder (ADHD) is a prevalent neurodevelopmental condition characterized by
age-inappropriate and impairing inattention and/or impulsiveness-hyperactivity based on Diagnostic and
Statistical Manual of Mental Disorders, fifth edition (DSM-5) and International Classification of Diseases (ICD-
10)(American Psychiatric Association, 2013). ADHD is commonly associated with school/academic,
occupational, and social dysfunction (Biederman, 2005; Caye et al., 2016). Furthermore, deficit in several
cognitive domains has been demonstrated in individuals with ADHD by neuropsychological studies (Sonuga-
Barke et al., 2010) In addition to neuropsychological, genetics and neurochemical studies investigating the
pathophysiology of ADHD (Caye et al., 2016), a large number of neuroimaging studies have been published
over the last three decades to elucidate potential structural or functional alterations of ADHD (Hoogman et al.,
2017; Konrad and Eickhoff, 2010). However, their findings are often inconsistent or conflicting. Hence, based
on these individual studies, the neural correlates of ADHD remain elusive.
Coordinate based meta-analysis (CBMA) of neuroimaging experiments can overcome these issues by
providing a synoptic view of findings across studies, hereby consolidating the existing literature (Eickhoff et al.,
2012; Turkeltaub et al., 2002). CBMA uses the reported peak coordinates to identify “if” and “where” the
convergence between reported coordinates is higher than expected by chance (Eickhoff et al., 2012). Previous
meta-analyses on neuroimaging studies in ADHD have focused on structural imaging (Ellison-Wright et al.,
2008; Frodl and Skokauskas, 2012; Nakao et al., 2011; Valera et al., 2007) or task-based functional imaging
only (Cortese et al., 2012; Dickstein et al., 2006; Hart et al., 2012; Hart et al., 2013; Lei et al., 2015; McCarthy
et al., 2014) (Supplemental Table S1). In addition of being outdated, however, they also yielded inconsistent
findings. Meta-analyses of structural imaging studies suggested regional gray matter volume reductions in
various cortical regions and also the basal ganglia and cerebellum, but showed limited agreement across
analyses (Ellison-Wright et al., 2008; Frodl and Skokauskas, 2012; Nakao et al., 2011; Valera et al., 2007).
Likewise, meta-analyses of activation studies heterogeneously indicated aberrations in a wide number of
regions covering different cerebral lobes and systems as well as the basal ganglia, thalamus and cerebellum
(Cortese et al., 2012; Dickstein et al., 2006; Hart et al., 2012; Hart et al., 2013; Lei et al., 2015; McCarthy et al.,
2014).
Divergence across previous meta-analyses may in part reflects rather low number of included studies,
ACCEPTED MANUSCRIP
T
5
different in-/exclusion criteria, (too) liberal statistical thresholding in search for positive findings (Muller et al.,
2018; Tahmasian M, 2018). Moreover, heterogeneity in the examined hypotheses, the assessed clinical
populations (e.g. subtype or severity of patients), the applied statistical approaches, and last but not least,
different practices in reporting negative findings related to the included individual studies are important factors
too (Muller et al., 2018; Tahmasian M, 2018). To address the heterogeneity and statistical power issues of the
previous meta-analyses, we conducted a large scale structural and functional meta-analysis, following recently
established consensus guidelines for neuroimaging meta-analyses suggested by the developers of all major
software packages (Muller et al., 2018). The current meta-analysis is based on the largest number of original
ADHD findings, strict adherence to best-practice protocols and stringent thresholding. The first aim of the
current study was to find spatially consistent brain abnormalities in patients with ADHD compared to healthy
comparisons. Hence, we pooled all the structural and functional studies, to provide a comprehensive overview
of grey matter and functional alterations in ADHD. This approach has been applied previously to other
neuropsychiatric disorder (Radua et al., 2012; Raschle et al., 2015; Sacher et al., 2012; Tahmasian et al.,
2016; Tahmasian M, 2018; Zakzanis et al., 2003). Moreover, with regard to heterogeneity issues, we
conducted several sub-analyses, clustered by extracted factors representing potential heterogeneity sources
(i.e. modality, medication status, gender or behavioral subtype ADHD patients as well as specific cognitive
domains and stimuli type of the tasks used in task-fMRI studies). By revisiting the issue of neuroimaging
findings in ADHD, we thus aim to settle the ongoing dispute on the presence and localization of structural
and/or functional brain alterations in children and adolescents with ADHD.
2. Methods
2.1. Search strategy and selection criteria
Based on the Preferred Reporting Items for Systematic Reviews and Meta-Analyses(Moher et al.) and
current consensus guidelines for neuroimaging meta-analyses (Muller et al., 2018), we searched PubMed,
OVID (EMBASE, ERIC and Medline), Web of Knowledge,and Scopus up to June 1,2018,for neuroimaging
studies on ADHD using the following search terms: (ADHD OR attention-deficit hyperactivity disorder)
AND ("functional magnetic resonance imaging" OR fMRI OR "voxel-based morphometry" OR VBM) AND
(children OR adolescents). In addition, we identified further papers by reference tracing and consulting
ACCEPTED MANUSCRIP
T
6
review articles. Next, two authors (FS and SS) independently screened all the identified abstracts. Case-
reports, letters to editors, reviews, meta-analyses, methodological studies and reports based on < 10
subjects per group were excluded as suggested previously (Muller et al., 2018; Tahmasian et al., 2017;
Tahmasian et al., 2016). Furthermore, we excluded all studies using region of interest (ROI) analysis, as
the null distribution in CBMA reflects a random spatial association between findings across the entire
brain. The assumption that each voxel has a priori the same chance for being reported, however, is
violated by ROI analyses, creating a sizable bias and inflated significance for the respective regions
(Muller et al., 2018; Muller et al., 2017).
Diagnosis of ADHD patients in the included papers had to be based on DSM-IV-TR, or DSM-5, or
ICD-10 criteria. The other criteria include the mean age < 18 year old, no neurological or psychiatric
comorbidities (such as depression, anxiety, autism, learning disorder, and epilepsy) or IQ > 70. We only
included the experiments performing a group comparison between patients with ADHD and healthy
controls (i.e., no within-group contrasts or contrasts of patients with ADHD versus patients with other
disorders). We did not exclude reports on medicated ADHD patients in order to reflect the fact that these
represent the bulk of the current literature. However, all included patients were off-medicated during the
image acquisition. As suggested (Muller et al., 2018; Muller et al., 2017), studies reporting the effects of
pharmacologic or psychological treatment were only included in case the authors reported between-group
differences at baseline or main effects of diagnosis.
2.2. Organization of Coordinates
Extracted data included bibliographic information, age, gender & number of subjects, imaging modality,
subtype and medication status of patients with ADHD, and the peak coordinates of group comparisons in
Montreal Neurological Institute (MNI) (A.C. Evans, 1993) or Talairach (Talairach and Tournoux, 1988)
space with the latter being subsequently transformed into MNI space.(Lancaster et al., 2007) Of note, in
neuroimaging meta-analyses, “study” refers to a single scientific publication while “experiment” denotes a
particular comparison, i.e., a contrast yielding a distinct set of coordinates.
Given that the samples of several studies (partially) overlapped, including such experiments may
yield spurious convergence. Thus, we followed the recommended approach to organize data by subjects
ACCEPTED MANUSCRIP
T
7
rather than experiments, avoiding undue influence of a patient cohort (Turkeltaub et al., 2012).
Specifically, we combined experiments in the same direction (in-/decreases) for a given set of patients,
yielding a maximum of two “experiments” per sample consisting of all extracted coordinates for this set of
patients (i.e., one for ADHD > Control contrast, one for Control > ADHD contrast). Of note, the
experiments were merged regardless of whether separate experiments were reported in one or different
papers whenever identity of the subjects could be established (Turkeltaub et al., 2012).
2.3. Activation likelihood estimation
We used the revised version of the Activation Likelihood Estimation (ALE) algorithm (Eickhoff et al., 2012)
to test significant convergence between activation foci relative to a null-hypothesis of random spatial
association between experiments. Firstly, the reported foci were modeled as center peaks of 3D Gaussian
probability distributions representing the spatial uncertainty associated with these arising from both
sampling effects and methodological differences in data processing and analysis. Importantly, the
modeled uncertainty is scaled by the number of subjects in the smaller group to accommodate higher
uncertainty of findings from smaller samples. The ensuing per-experiment “modeled activation” maps were
then combined into an ALE map by computing their union across experiments (Eickhoff et al., 2012;
Turkeltaub et al., 2012). Subsequently, an analytical approach based on non-linear histogram integration
was conducted to test against the null hypothesis of random spatial association (Eickhoff et al., 2012;
Turkeltaub et al., 2012), followed by cluster-level family-wise error (cFWE) correction at p < 0.05 (cluster-
forming threshold of p < 0·001 on the voxel level) based on Monte-Carlo simulation (Eickhoff et al., 2017;
Muller et al., 2018).
2.4. Performed analyses
We first performed an ALE analysis across all identified experiments in order to probe for abnormalities
independently of direction and neuroimaging modality. Next, we performed three separate sets of
analyses assessing increases, decreases or aberrations (pooling in-/decreases) in structural (VBM) and
functional (fMRI) imaging, respectively. As recommended, separate analyses were performed only for
those combinations of direction and modality yielding at least 17 experiments, given consideration on
ACCEPTED MANUSCRIP
T
8
robustness and power (Eickhoff et al., 2016). Hence, specific analysis of rs-fMRI studies was not possible
due to low number of experiments. Relatedly, we also split the available experiments by medication
status, gender or behavioral subtype ADHD patients, noting that the number of experiments for female
patients as well as isolated inattentive or hyperactive subtypes was insufficient for analysis.
Furthermore, we probed for convergence among task-fMRI studies when focusing only on specific
cognitive domains and found a sufficient number of experiments (i.e., > 17) (Eickhoff et al., 2016) for
inhibitory and attention, but also performed a joint analyses on the remaining cognitive tasks (memory,
timing, reasoning). Number of experiments on reward processing tasks, were not sufficient to analyze.
Finally, the available studies were also categorized by stimulus type (emotion, reward, neutral), but only
field a sufficient number for a sub-analysis of neutral stimuli.
Finally, for significant convergent findings, related to subgroups of experiments e.g. subgroup of all
fMRI experiments representing male only, we checked the number of studies, contributed to the
convergent cluster. The question was whether the contribution rate represents the proportion of all related
experiments in the database. For example, if X out of P fMRI experiments with mail only contribute to a
convergent cluster, we evaluated that how likely it is that, this contribution rate represent proportion of P
fMRI experiments across all available experiments (96) in the database. We tested this for each significant
finding separately, using binomial test in SPSS (v22). Wherever the contribution rate of a subgroup of
experiments represents the proportion of their experiments across all experiments in the database, one
could infer that the convergent finding may be driven by the effects of main indicator (e.g. male only) of the
subgroup.
Results
From a pool of 1586 retrieved publications, 205 potentially eligible studies were identified. Among them,
109 were subsequently excluded due to reasons including ROI analyses, no reported contrast between
ADHD and healthy subjects, or reporting of longitudinal effects without baseline. In case the study met all
the criteria except reporting the peak coordinates for group comparisons, we contacted the authors to
obtain the relevant information. In total, 96 studies were finally eligible for the current meta-analyses
(Figure 1, Supplemental Table S2) reporting a total of 130 individual experiments (92 task-fMRI, 32 VBM
ACCEPTED MANUSCRIP
T
9
and 6 rs-fMRI; Supplement) based on 1914 unique subjects. Of these, 62.7% reported decreases in
ADHD patients compared to controls (54 task-fMRI, 25 VBM and 3 rs-fMRI; Supplement).
2.5. Meta-analyses of neuroimaging findings in ADHD
Overall, we conducted several separate ALE meta-analyses. When pooling in-/decreases experiments
(Figure 2), the results yielded p= 0.129 (across structural and functional findings), p= 0.452 (for VBM
only), and p= 0.212 (for fMRI, pooling across task and resting state). Also a restriction to task-fMRI
experiments did not provide a significance finding (p= 0.062). Similarly, restricting meta-analyses to in-
/decreases in ADHD, lead to non-significant findings (decreased: all p= 0.195, VBM p= 0.341, fMRI p=
0.138, task-fMRI only p= 0.329; increased: all p= 0.175; fMRI p= 0.153, task-fMRI p= 0.668; Table 1A). Of
note, repeating all analyses with an alternative, potentially more liberal statistical threshold (i.e., threshold-
free cluster enhancement, TFCE (Smith and Nichols, 2009)), fully corroborated these results and likewise
reveal non-significant convergence (Table 1A).
2.6. Follow-up sub-analyses on task fMRI findings
Of the 68 task-fMRI studies, 33% investigated inhibitory control, 29% attention, 25% other cognitive
domains and 13% reward processing (Supplement). With respect to stimulus type, 70% used neutral, 12%
emotional and 17% rewarding stimuli (Supplement). Again, no significant convergence (Table 1B) was
found in any of the more restricted and hence specific sub-analyses for which a sufficient number of
experiments was available (inhibitory control p= 0.302, attention p= 0.847, all other cognitive domains p=
0.520), even though we noted a trend towards significance when specifically analyzing the convergence of
decreased activity for inhibitory control (p= 0.052). Repeating all analyses with TFCE threshold, the latter
result did pass statistical significance at p= 0.040 (Table 1B).
We also conducted separate analyses on in-/decreases and their pooled effect for only those experiments
using neutral stimuli and found significant aberrant convergence in the left pallidum/putamen (local
maximum: -18, 4, -4 in MNI space, 109 voxels, 69.7% left Pallidum, 12.7% left Putamen) when looking at
ACCEPTED MANUSCRIP
T
10
the pooled in-/decreased activation experiments in ADHD (p= 0.036), but not for either direction
individually (decrease p= 0.244, increase p= 0.110 (Figure 3A, Table 1C).
Finally, we also repeated all main and follow-up analyses including only medication-naïve, male or
combined-type ADHD patients, respectively, yielding a single (marginally significant) convergence for
decreased fMRI (task-fMRI and rs-fMRI) in male subjects on the left inferior frontal gyrus (IFG) (local
maximum: -38, 26, -16 in MNI space, 93 voxels, 83% in frontal orbital cortex) (p=0.049, Figure 3B,
Supplement). Moreover, we used binomial test on the contribution-rate (i.e. 0.13 (4 out of 30) of
decreased fMRI experiments with male only and their main proportion in the database (i.e.52% (30 out of
57 decreased fMRI experiments). The result indicated that observed contribution-rate significantly is less
than the chance probability (observed-prob= 0.13, test-prob= 0.52, p-value= 0.001). Regarding the left
Putamen-Pallidum, 11 out of 41 task-fMRI experiments with neutral stimuli, contributed to this convergent
finding, while the proportion of task-fMRI experiments with neutral stimuli in the database was 0.44 (41out
of 92 task-fMRI experiments). The result of binomial test was not significant (observed-prob= 0.37, test-
prob= 0.44, p-value= 0.268).
Discussion
The current study provides the largest and most comprehensive meta-analytic summary of neuroimaging
findings in children and adolescents with ADHD across several imaging modalities, following the current
best-practice recommendations (Muller et al., 2018). The pooled structural and functional assessment and
the additional sub-analyses focusing on homogeneous subsets of experiments (in terms of modality and
direction) yielded no significant convergence across the available ADHD literature. Reasons of this
heterogeneity may be accounted for several issues including clinical heterogeneity and variations in
experimental design and analysis. We discuss further this heterogeneity in the next section. Moreover, we
also restricted all the experiments by medication-status, gender, ADHD sub-types, as well as task-fMRI by
specific cognitive domains and stimulus types, and observed convergence aberrant finding in the task-
ACCEPTED MANUSCRIP
T
11
fMRI experiments (using neutral stimuli) in the left pallidum/putamen and decreased activity (in male
subjects only) in the left IFG. Dysfunction of the pallidum/putamen and IFG has been reported in ADHD
previously (Castellanos and Proal, 2012; Cortese et al., 2012; Hart et al., 2012; Hart et al., 2013; Konrad
and Eickhoff, 2010; Rubia, 2011), which is in line with the fronto-striatal pathway dysfunction model of
ADHD. The dopamine-rich ventral putamen receives fibers from the medial orbitofrontal cortex and IFG
and it has been shown that their abnormality is linked with hyperactivity, impulsivity, disinhibition, and
inattention symptoms of ADHD (Itami and Uno, 2002; Max et al., 2002; Rubia, 2011).
2.7. Neuroimaging in ADHD: A heterogeneous field
The heterogeneity in experimental design and procedure across the task-based fMRI experiments, which
containing 70% of our included studies, might be one of the potential sources of inconsistent results. Most
available tasks were often aimed at probing various functional impairments in children/adolescents with
ADHD (Arnsten and Rubia, 2012). The paradigms of included tasks varied widely (i.e. Go/No-Go, Stroop,
Stop signal, timing, reward processing, memory and attention tasks), but each of them applied in less than
10 studies. In addition to the paradigm diversity, there is flexibility in each paradigm regarding to the type
of stimuli, presentation of stimuli and type of trials. Due to these inconsistencies, the cognitive operations
performed by the subjects when engaging in the respective tasks should vary widely. From the overall
inconsistent results of the current study, we conclude that there is limited convergent regional abnormality
that was tapped into by the structural and functional experiments that have been used to study ADHD, to
date. Additionally, across cognitive domains, there is no convergence in the neurobiological aberrations
underlying ADHD. Worthy of note, in the structural imaging studies (i.e. VBM), experimental or design
flexibility is absent, but analytical heterogeneity still plays a major role (e.g., various statistical thresholds
or spatial transformation of the images). Finally, the included rs-fMRI analytical approaches were likewise
vastly different. This methodological multiplicity may have contributed a lot of variance to the current
literature beyond what could already be expected given the heterogeneity of the clinical presentation in
ADHD.
Our divergent results on structural and functional experiments support the view that ADHD is a
multi-faceted disorder, which affects several functional and behavioral domains. We would hence side with
ACCEPTED MANUSCRIP
T
12
the hypothesis, that ADHD may be primarily reflecting a dysconnectivity disorder, i.e., that the various
cognitive and affective affections in ADHD patients may stem from impaired communication and
integration between the brain regions/networks rather than from regional abnormalities only (Castellanos
and Proal, 2012; Konrad and Eickhoff, 2010). Of note, here we could not test the network alterations due
to low number of available rs-fMRI experiment. Also, as recommended, we did not include the ROI-based
functional connectivity publications (Muller et al., 2018). In particular, such distributed network pathology
would explain both the lack of convergence in either structural or functional imaging findings, as well as
the heterogeneous expression of the core and associated symptoms across patients. The latter is
particularly important, as ADHD is clinically described as a heterogeneous disorder, which includes
impairment on several cognitive and emotional domains (Wahlstedt et al., 2009). These deficits have
differential influence on neuropsychological functioning of patients and lead to various clinical
presentations (Wahlstedt et al., 2009). Although ADHD is often classified into three different presentations,
they have considerable overlap (Wahlstedt et al., 2009). A recent neuroimaging study also found no
common brain abnormalities in all ADHD subgroups, suggesting that ADHD is a collection of discrete
disorders (Stevens et al., 2018). It is worth mentioning that most of the included studies either recruited
patients from different presentations or did not specify the clinical presentation of ADHD that was
investigated, even though diagnostic validity of such presentation classification is questionable per se
(Bernfeld, 2012). Moreover, most studies retained in the present meta-analysis included participants with
high rates of comorbidities, which increases the clinical heterogeneity of the samples (Elia et al., 2008).
Thus, even though all patients were labeled as ADHD, they may have presented quite differently, both
within each and across samples, potentially featuring different underlying pathologies and hereby further
contributing to the lack of convergence using combination of structural and functional experiments. We
discuss now key methodological issue form the body of research that we included.
2.8. Methodological issues
A main advantage of the ALE meta-analysis approach is integration of a large number of findings to
establish consensus on the spatial locations of regional disruptions in neuropsychiatric disorders (Eickhoff
et al., 2012; Goodkind et al., 2015; Muller et al., 2017; Tahmasian et al., 2017; Tahmasian et al., 2018;
ACCEPTED MANUSCRIP
T
13
Tahmasian et al., 2016). Thus, ALE allows synthesizing the multitude of single studies in an unbiased
fashion and consolidates the available literature, overcoming problems associated with individual
neuroimaging experiments (Eickhoff et al., 2012; Muller et al., 2018). Here, we followed the recently
developed best-practice protocols of neuroimaging meta-analysis (Muller et al., 2018). In particular, we
performed multiple-comparison correction using the cluster-level FWE correction, which should be
preferred over the liberal but previously popular false discovery rate (FDR) to provide maximum statistical
rigor (Eickhoff et al., 2017; Eickhoff et al., 2016).
In addition, it has been suggested to include at least 17 experiments into an ALE meta-analysis to
achieve 80% power for at least moderate to strong effects (Eickhoff et al., 2016). To explore the potential
moderators that might influence on our results, we first identified all eligible experiments and
subsequently, categorized them by available variety sources including imaging modality, medication
status, gender or behavioral subtype ADHD patients. However, the number of experiments of most of the
categories (including rs-fMRI, as well as female patients and isolated inattentive or hyperactive subtypes)
were insufficient for a valid analysis. Given that ALE analyses with very few sets of experiments have
been shown to be unstable and potentially driven by individual studies, we could not perform the initially
planned set of assessments for the effects of most potential moderators. Indeed, exploring moderators is
not feasible unless each subgroup yields enough experiments for a separate meta-analysis. Furthermore,
by splitting the task-fMRI experiments with specific cognitive domains and also stimulus type of the tasks,
the issue was the same, as sufficient number of experiments were not supplied for all subgroups.
Accordingly, we could only perform separate meta-analyses for individual subgroups of experiments,
categorized by inhibitory, attention, and also joint of the remaining cognitive tasks (memory, timing, and
reasoning), as well as neutral stimuli, to probe for convergent functional abnormalities in children and
adolescents with ADHD. However, the results were significant only for two subgroups of experiments, i.e
task-fMRI experiments with neutral stimuli and decreased fMRI experiments with male only. According to
the result of binomial test for neutral stimuli experiments, the contribution-rate of experiments represents
the main proportion of the experiments with neutral stimuli in the database. Hence, the convergent finding
of task-fMRI experiments may be driven by the effects of using tasks with neutral stimuli. Moreover,
regarding the decreased fMRI experiments with male only, the result of the binomial test indicated that
ACCEPTED MANUSCRIP
T
14
observed contribution-rate significantly is less than the chance probability. Hence, the contribution-rate of
experiments with male only is likely an under-representation of the expected proportion in the database.
Moreover, we carefully excluded studies with ROI analysis to avoid inflating significance for the
particular regions (Muller et al., 2018). Importantly, we organized our dataset based on the recommended
approach, which has been introduced to minimize within-group effects (Turkeltaub et al., 2012) We
discuss now our findings in the light of previous ADHD meta-analyses.
2.9. Divergence of previous mega- and meta-analyses findings
Previous ADHD meta-analyses focusing on structural imaging (Ellison-Wright et al., 2008; Frodl and
Skokauskas, 2012; Nakao et al., 2011; Valera et al., 2007) and task-based fMRI (Cortese et al., 2012;
Dickstein et al., 2006; Hart et al., 2012; Hart et al., 2013; Lei et al., 2015; McCarthy et al., 2014) found
significant brain abnormalities in variety of brain regions (Supplemental Table S1). Hence, not only
individual studies, but also previous meta-analyses in children/adolescents with ADHD have yielded
inconsistent findings. This might be due to the fact that those meta-analyses were not following the current
best-practice guideline, which is developed recently (Muller et al., 2018). Results of such meta-analyses
may be in part driven by small sample size, liberal thresholding and other methodological choices that
should be considered non-optimal currently. For example, three meta-analyses on task fMRI (Cortese et
al., 2012; Dickstein et al., 2006; Lei et al., 2015) and one on VBM studies (Ellison-Wright et al., 2008)
used a version of GingerALE, which was later discovered to have a bug leading to inadequately liberal
thresholds that were substantially different from the requested level and did not control for multiple
comparisons (Eickhoff et al., 2017). In turn, two fMRI meta-analyses focusing on timing (Hart et al., 2012)
and inhibition/attention (Hart et al., 2013), as well as two VBM meta-analyses applied the Signed
Differential Mapping (SDM) method (Frodl and Skokauskas, 2012; Nakao et al., 2011), which by design
are being more liberal, i.e., to err on the side of false positives rather than negatives. Last but not least,
previous meta-analyses assessed substantially smaller samples of experiments, which entail a high
likelihood (formally) significant convergence might be often driven by few experiments and dominates the
regional effects of those studies (Muller et al., 2018). Given the current growth of the literature, however,
we were able to apply more stringent in-/exclusion criteria and still analyze an unprecedentedly large
ACCEPTED MANUSCRIP
T
15
number of individual experiments. Our included studies are overlapping with those of previous meta-
analyses in various degrees. We excluded the ones that did not meet our inclusion criteria (Supplemental
Table S1).
Although there are many available CBMA with significant consistent results in various
neuropsychiatric disorders (Cortese et al., 2016; Cortese et al., 2012; Goodkind et al., 2015; Herz et al.,
2014; Radua et al., 2012; Tahmasian et al., 2017; Tahmasian et al., 2016; Wegbreit et al., 2014) , some
previous meta-analyses in unipolar depression (Muller et al., 2017) and insomnia disorder (Tahmasian et
al., 2018; Tahmasian M, 2018) investigated a large body of literature and followed the best-practice
guideline (i.e. rigorous in-/exclusion criteria and statistical threshold), but still observed a lack of
convergence across their sub-analyses. The authors suggested that small samples of participants, clinical
variability, various experimental design, flexibility in preprocessing, and statistical approaches could be the
possible reasons of observed heterogeneity in such disorder (Muller et al., 2017; Tahmasian et al., 2018).
Recently, a study comprising 1713 participants with ADHD and 1529 controls from 23
sites applied FreeSurfer and found that volume of the accumbens, amygdala, caudate, hippocampus,
putamen, and intracranial volume were smaller in subjects with ADHD compared with controls (9). We did
not include this paper, as they focused on particular subcortical ROIs, which could affect the whole brain
results. In the present study, we included 28 structural experiments which yielded no significant volume
alterations. Of note, the mentioned study has a unified analysis protocol and therefore has less
experimental and analytical noise and hence is more likely to find the regional abnormality. However, it is
not clear whether the findings are just reflecting their particular regional choices or are robust across
studies, which can be tested by neuroimaging meta-analyses.
2.10. Future directions
Technical problems and clinical heterogeneity aside, we would argue that one of the major obstacles in
neuroimaging research is the strong bias towards publishing positive results, combined with low sample
size and high preprocessing and analytical flexibility (garden of forking paths) in (f)MRI analyses. It stands
to reason that this combination provides the wrong incentives to analyze data in various ways until
significant results emerge, which are often spurious and not replicable or convergent. Coupled with the
ACCEPTED MANUSCRIP
T
16
fact that direct replication studies are still rare, we would thus worry that a large body of the imaging
literature contains inflated reports of regional effects which only becomes apparent once well-powered and
robust (through their size) meta-analyses as the current are performed. To us, this indicates a need for
change at several levels including higher-powered original studies, homogenous clinical populations, pre-
registration of analysis plans, a better standardization of processing pipelines (also from the level of
replicability), large scale open data sharing, and maybe most importantly a cultural shift away from the
current bias towards more likely publication of positive findings as well-powered inconsistent findings may
be as important to move the entire field forward.
3. Conclusion
To the best of our knowledge, this study is the largest meta-analysis of structural and functional
neuroimaging experiments in children/adolescents with ADHD. We found no significant convergent across
structural and functional regional alterations in ADHD, which might be attributable to clinical heterogeneity,
experimental and analytical flexibility and positive publication bias, but could also point towards a more
distributed, network-based pathology lacking a consistent expression at any particular location. Among
many different sub-analyses, we only observed the convergence dysfunction for task-fMRI experiments
(using neutral stimuli) in the left pallidum/putamen and decreased activity (using male subjects) in the left
IFG. This study highlights the need for further exploration assessing regional structural and functional
maladaptation, as well as connectivity in parallel to unravel the relation between abnormal regional effects
and disturbed integration in ADHD.
Conflict of interest
The authors declare that the research was conducted in the absence of any commercial or financial
relationships that could be construed as a potential conflict of interest.
Acknowledgements
SBE is supported by the Deutsche Forschungsgemeinschaft DFG (EI 816/11-1), the National Institute of
Mental Health (R01-MH074457), the Helmholtz Portfolio Theme "Supercomputing and Modeling for the
ACCEPTED MANUSCRIP
T
17
Human Brain" and the European Union’s Horizon 2020 Research and Innovation Programme under Grant
Agreement No. 785907 (HBP SGA2).
ACCEPTED MANUSCRIP
T
18
References
A.C. Evans, D.L.C., S.R Mills, E.D. Brown, R.L. Kelly, T.M. Peters, 1993. 3D statistical neuroanatomical models from 305 MRI volumes. Nuclear Science Symposium and Medical Imaging Conference, IEEE Conference Record, 1813–1817.
American Psychiatric Association, 2013. Diagnostic and Statistical Manual of Mental Disorders, 5th ed, Washington, DC.
Arnsten, A.F., Rubia, K., 2012. Neurobiological circuits regulating attention, cognitive control, motivation, and emotion: disruptions in neurodevelopmental psychiatric disorders. J Am Acad Child Adolesc Psychiatry 51, 356-367.
Bernfeld, J., 2012. ADHD and factor analysis: are there really three distinct subtypes of ADHD? Appl Neuropsychol Child 1, 100-104.
Biederman, J., 2005. Attention-deficit/hyperactivity disorder: a selective overview. Biol Psychiatry 57, 1215-1220.
Castellanos, F.X., Proal, E., 2012. Large-scale brain systems in ADHD: beyond the prefrontal-striatal model. Trends Cogn Sci 16, 17-26.
Caye, A., Rocha, T.B., Anselmi, L., Murray, J., Menezes, A.M., Barros, F.C., Goncalves, H., Wehrmeister, F., Jensen, C.M., Steinhausen, H.C., Swanson, J.M., Kieling, C., Rohde, L.A., 2016. Attention-Deficit/Hyperactivity Disorder Trajectories From Childhood to Young Adulthood: Evidence From a Birth Cohort Supporting a Late-Onset Syndrome. JAMA Psychiatry 73, 705-712.
Cortese, S., Castellanos, F.X., Eickhoff, C.R., D'Acunto, G., Masi, G., Fox, P.T., Laird, A.R., Eickhoff, S.B., 2016. Functional Decoding and Meta-analytic Connectivity Modeling in Adult Attention-Deficit/Hyperactivity Disorder. Biol Psychiatry 80, 896-904.
Cortese, S., Kelly, C., Chabernaud, C., Proal, E., Di Martino, A., Milham, M.P., Castellanos, F.X., 2012. Toward systems neuroscience of ADHD: a meta-analysis of 55 fMRI studies. Am J Psychiatry 169, 1038-1055.
Dickstein, S.G., Bannon, K., Castellanos, F.X., Milham, M.P., 2006. The neural correlates of attention deficit hyperactivity disorder: an ALE meta-analysis. J Child Psychol Psychiatry 47, 1051-1062.
Eickhoff, S.B., Bzdok, D., Laird, A.R., Kurth, F., Fox, P.T., 2012. Activation likelihood estimation meta-analysis revisited. Neuroimage 59, 2349-2361.
Eickhoff, S.B., Laird, A.R., Fox, P.M., Lancaster, J.L., Fox, P.T., 2017. Implementation errors in the GingerALE Software: Description and recommendations. Hum Brain Mapp 38, 7-11.
Eickhoff, S.B., Nichols, T.E., Laird, A.R., Hoffstaedter, F., Amunts, K., Fox, P.T., Bzdok, D., Eickhoff, C.R., 2016. Behavior, sensitivity, and power of activation likelihood estimation characterized by massive empirical simulation. Neuroimage 137, 70-85.
Elia, J., Ambrosini, P., Berrettini, W., 2008. ADHD characteristics: I. Concurrent co-morbidity patterns in children & adolescents. Child Adolesc Psychiatry Ment Health 2, 15.
Ellison-Wright, I., Ellison-Wright, Z., Bullmore, E., 2008. Structural brain change in Attention Deficit Hyperactivity Disorder identified by meta-analysis. BMC psychiatry 8, 51.
ACCEPTED MANUSCRIP
T
19
Frodl, T., Skokauskas, N., 2012. Meta-analysis of structural MRI studies in children and adults with attention deficit hyperactivity disorder indicates treatment effects. Acta psychiatrica Scandinavica 125, 114-126.
Goodkind, M., Eickhoff, S.B., Oathes, D.J., Jiang, Y., Chang, A., Jones-Hagata, L.B., Ortega, B.N., Zaiko, Y.V., Roach, E.L., Korgaonkar, M.S., Grieve, S.M., Galatzer-Levy, I., Fox, P.T., Etkin, A., 2015. Identification of a common neurobiological substrate for mental illness. JAMA Psychiatry 72, 305-315.
Hart, H., Radua, J., Mataix-Cols, D., Rubia, K., 2012. Meta-analysis of fMRI studies of timing in attention-deficit hyperactivity disorder (ADHD). Neurosci Biobehav Rev 36, 2248-2256.
Hart, H., Radua, J., Nakao, T., Mataix-Cols, D., Rubia, K., 2013. Meta-analysis of functional magnetic resonance imaging studies of inhibition and attention in attention-deficit/hyperactivity disorder: exploring task-specific, stimulant medication, and age effects. JAMA Psychiatry 70, 185-198.
Herz, D.M., Eickhoff, S.B., Lokkegaard, A., Siebner, H.R., 2014. Functional neuroimaging of motor control in Parkinson's disease: a meta-analysis. Hum Brain Mapp 35, 3227-3237.
Hoogman, M., Bralten, J., Hibar, D.P., Mennes, M., Zwiers, M.P., Schweren, L.S.J., van Hulzen, K.J.E., Medland, S.E., Shumskaya, E., Jahanshad, N., Zeeuw, P., Szekely, E., Sudre, G., Wolfers, T., Onnink, A.M.H., Dammers, J.T., Mostert, J.C., Vives-Gilabert, Y., Kohls, G., Oberwelland, E., Seitz, J., Schulte-Ruther, M., Ambrosino, S., Doyle, A.E., Hovik, M.F., Dramsdahl, M., Tamm, L., van Erp, T.G.M., Dale, A., Schork, A., Conzelmann, A., Zierhut, K., Baur, R., McCarthy, H., Yoncheva, Y.N., Cubillo, A., Chantiluke, K., Mehta, M.A., Paloyelis, Y., Hohmann, S., Baumeister, S., Bramati, I., Mattos, P., Tovar-Moll, F., Douglas, P., Banaschewski, T., Brandeis, D., Kuntsi, J., Asherson, P., Rubia, K., Kelly, C., Martino, A.D., Milham, M.P., Castellanos, F.X., Frodl, T., Zentis, M., Lesch, K.P., Reif, A., Pauli, P., Jernigan, T.L., Haavik, J., Plessen, K.J., Lundervold, A.J., Hugdahl, K., Seidman, L.J., Biederman, J., Rommelse, N., Heslenfeld, D.J., Hartman, C.A., Hoekstra, P.J., Oosterlaan, J., Polier, G.V., Konrad, K., Vilarroya, O., Ramos-Quiroga, J.A., Soliva, J.C., Durston, S., Buitelaar, J.K., Faraone, S.V., Shaw, P., Thompson, P.M., Franke, B., 2017. Subcortical brain volume differences in participants with attention deficit hyperactivity disorder in children and adults: a cross-sectional mega-analysis. Lancet Psychiatry 4, 310-319.
Itami, S., Uno, H., 2002. Orbitofrontal cortex dysfunction in attention-deficit hyperactivity disorder revealed by reversal and extinction tasks. Neuroreport 13, 2453-2457.
Konrad, K., Eickhoff, S.B., 2010. Is the ADHD brain wired differently? A review on structural and functional connectivity in attention deficit hyperactivity disorder. Hum Brain Mapp 31, 904-916.
Lancaster, J.L., Tordesillas-Gutierrez, D., Martinez, M., Salinas, F., Evans, A., ZilleS, K., Mazziotta, J.C., Fox, P.T., 2007. Bias between MNI and talairach coordinates analyzed using the ICBM-152 brain template. Human Brain Mapping 28, 1194-1205.
Lei, D., Du, M., Wu, M., Chen, T., Huang, X., Du, X., Bi, F., Kemp, G.J., Gong, Q., 2015. Functional MRI reveals different response inhibition between adults and children with ADHD. Neuropsychology 29, 874-881.
Max, J.E., Fox, P.T., Lancaster, J.L., Kochunov, P., Mathews, K., Manes, F.F., Robertson, B.A., Arndt, S., Robin, D.A., Lansing, A.E., 2002. Putamen lesions and the development of attention-deficit/hyperactivity symptomatology. J Am Acad Child Adolesc Psychiatry 41, 563-571.
McCarthy, H., Skokauskas, N., Frodl, T., 2014. Identifying a consistent pattern of neural function in attention deficit hyperactivity disorder: a meta-analysis. Psychol Med 44, 869-880.
Moher, D., Liberati, A., Tetzlaff, J., Altman, D.G., Group, P., 2009. Preferred reporting items for systematic reviews and meta-analyses: the PRISMA statement. PLoS Med 6, e1000097.
ACCEPTED MANUSCRIP
T
20
Muller, V.I., Cieslik, E.C., Laird, A.R., Fox, P.T., Radua, J., Mataix-Cols, D., Tench, C.R., Yarkoni, T., Nichols, T.E., Turkeltaub, P.E., Wager, T.D., Eickhoff, S.B., 2018. Ten simple rules for neuroimaging meta-analysis. Neurosci Biobehav Rev 84, 151-161.
Muller, V.I., Cieslik, E.C., Serbanescu, I., Laird, A.R., Fox, P.T., Eickhoff, S.B., 2017. Altered Brain Activity in Unipolar Depression Revisited: Meta-analyses of Neuroimaging Studies. JAMA Psychiatry 74, 47-55.
Nakao, T., Radua, J., Rubia, K., Mataix-Cols, D., 2011. Gray matter volume abnormalities in ADHD: voxel-based meta-analysis exploring the effects of age and stimulant medication. Am J Psychiatry 168, 1154-1163.
Radua, J., Borgwardt, S., Crescini, A., Mataix-Cols, D., Meyer-Lindenberg, A., McGuire, P.K., Fusar-Poli, P., 2012. Multimodal meta-analysis of structural and functional brain changes in first episode psychosis and the effects of antipsychotic medication. Neurosci Biobehav Rev 36, 2325-2333.
Raschle, N.M., Menks, W.M., Fehlbaum, L.V., Tshomba, E., Stadler, C., 2015. Structural and Functional Alterations in Right Dorsomedial Prefrontal and Left Insular Cortex Co-Localize in Adolescents with Aggressive Behaviour: An ALE Meta-Analysis. PLoS One 10, e0136553.
Rubia, K., 2011. "Cool" inferior frontostriatal dysfunction in attention-deficit/hyperactivity disorder versus "hot" ventromedial orbitofrontal-limbic dysfunction in conduct disorder: a review. Biol Psychiatry 69, e69-87.
Sacher, J., Neumann, J., Funfstuck, T., Soliman, A., Villringer, A., Schroeter, M.L., 2012. Mapping the depressed brain: a meta-analysis of structural and functional alterations in major depressive disorder. J Affect Disord 140, 142-148.
Smith, S.M., Nichols, T.E., 2009. Threshold-free cluster enhancement: Addressing problems of smoothing, threshold dependence and localisation in cluster inference. Neuroimage 44, 83-98.
Sonuga-Barke, E., Bitsakou, P., Thompson, M., 2010. Beyond the dual pathway model: evidence for the dissociation of timing, inhibitory, and delay-related impairments in attention-deficit/hyperactivity disorder. J Am Acad Child Adolesc Psychiatry 49, 345-355.
Stevens, M.C., Pearlson, G.D., Calhoun, V.D., Bessette, K.L., 2018. Functional Neuroimaging Evidence for Distinct Neurobiological Pathways in Attention-Deficit/Hyperactivity Disorder. Biol Psychiatry Cogn Neurosci Neuroimaging 3, 675-685.
Tahmasian, M., Eickhoff, S.B., Giehl, K., Schwartz, F., Herz, D.M., Drzezga, A., van Eimeren, T., Laird, A.R., Fox, P.T., Khazaie, H., Zarei, M., Eggers, C., Eickhoff, C.R., 2017. Resting-state functional reorganization in Parkinson's disease: An activation likelihood estimation meta-analysis. Cortex 92, 119-138.
Tahmasian M, N.K., Samea F, Zarei M, Spiegelhalder K, Eickhoff SB, Van Someren E, Khazaie H, Eickhoff CR., 2018. Reply to Hua Liu, HaiCun Shi and PingLei Pan: Coordinate based meta-analyses in a medium sized literature: considerations, limitations and road ahead. Sleep Medicine Reviews.
Tahmasian, M., Noori, K., Samea, F., Zarei, M., Spiegelhalder, K., Eickhoff, S.B., van Someren, E., Khazaie, H., Eickhoff, C.R., 2018. A Lack of consistent brain alterations in insomnia disorder: an activation likelihood estimation meta-analysis. Sleep Medicine Reviews.
Tahmasian, M., Rosenzweig, I., Eickhoff, S.B., Sepehry, A.A., Laird, A.R., Fox, P.T., Morrell, M.J., Khazaie, H., Eickhoff, C.R., 2016. Structural and functional neural adaptations in obstructive sleep apnea: an activation likelihood estimation meta-analysis. Neurosci Biobehav Rev.
Talairach, J., Tournoux, P., 1988. Co-planar stereotaxic atlas of the human brain : 3-dimensional proportional system : an approach to cerebral imaging. Georg Thieme, Stuttgart ; New York.
ACCEPTED MANUSCRIP
T
21
Turkeltaub, P.E., Eden, G.F., Jones, K.M., Zeffiro, T.A., 2002. Meta-analysis of the functional neuroanatomy of single-word reading: method and validation. Neuroimage 16, 765-780.
Turkeltaub, P.E., Eickhoff, S.B., Laird, A.R., Fox, M., Wiener, M., Fox, P., 2012. Minimizing within-experiment and within-group effects in Activation Likelihood Estimation meta-analyses. Hum Brain Mapp 33, 1-13.
Valera, E.M., Faraone, S.V., Murray, K.E., Seidman, L.J., 2007. Meta-analysis of structural imaging findings in attention-deficit/hyperactivity disorder. Biol Psychiatry 61, 1361-1369.
Wahlstedt, C., Thorell, L.B., Bohlin, G., 2009. Heterogeneity in ADHD: neuropsychological pathways, comorbidity and symptom domains. J Abnorm Child Psychol 37, 551-564.
Wegbreit, E., Cushman, G.K., Puzia, M.E., Weissman, A.B., Kim, K.L., Laird, A.R., Dickstein, D.P., 2014. Developmental meta-analyses of the functional neural correlates of bipolar disorder. JAMA Psychiatry 71, 926-935.
Zakzanis, K.K., Graham, S.J., Campbell, Z., 2003. A meta-analysis of structural and functional brain imaging in dementia of the Alzheimer's type: a neuroimaging profile. Neuropsychol Rev 13, 1-18.
ACCEPTED MANUSCRIP
T
22
Figures’ legends
Figure 1. Study selection strategy flow chart. ROI: region of Interest, task-fMRI: task-based functional
magnetic resonance imaging, rs-fMRI: resting-state functional magnetic resonance imaging, VBM: voxel-
based morphometry, ADHD: attention-deficit/hyperactivity disorder.
ACCEPTED MANUSCRIP
T
23
Figure 2. Distribution of the included coordinates in the current study. Included coordinates
reflecting structural/functional alterations in children/adolescents with ADHD compared to healthy
ACCEPTED MANUSCRIP
T
24
subjects. Rs-fMRI: resting-state functional magnetic resonance imaging; task-fMRI: task-based functional
magnetic resonance imaging; VBM: voxel-based morphometry.
Figure 3. Convergent findings of sub-analyses. A. Aberrant activity (green) in the left pallidum/putamen
(-18, 4, -4 MNI, 109 voxels, p= 0.036), based on task-based fMRI experiments (using neutral stimuli only);
B. Decreased activity (blue) in the left inferior frontal gyrus (-38, 26, -16 MNI, 93 voxels, p =0.049 based
on fMRI (task-fMRI & rs-fMRI) experiments (using male patients only).
ACCEPTED MANUSCRIP
T
25
ACCEPTED MANUSCRIP
T
26
Table 1: Results of conducted meta-analyses in children/adolescent with ADHD compared to
healthy subjects
A. Modality
Number of
Experiments Modalities Contrasts
P-value
TFCE cFWE
1 130 VBM, task-fMRI, rs-fMRI – 0·170 0·129
2 81 VBM, task-fMRI, rs-fMRI Control > ADHD 0·087 0·195
3 48 VBM, task-fMRI, rs-fMRI ADHD > Control 0·104 0·175
4 98 fMRI
(combination of task- and rs-fMRI) – 0·151 0·212
5 57 fMRI
(combination of task- and rs-fMRI) Control > ADHD 0·061 0·138
6 1 fMRI
(combination of task- and rs-fMRI) ADHD > Control 0·063 0·153
7 92 task-fMRI – 0·064 0·062
8 54 task-fMRI Control > ADHD 0·103 0·329
9 38 task-fMRI ADHD > Control 0·248 0·668
10 32 VBM – 0·817 0·452
11 25 VBM Control > ADHD 0·630 0·341
B· Cognitive domain
Number of
Experiments Domain Contrasts
P-value
TFCE cFWE
12 31 Inhibitory control – 0·405 0·302
13 18 Inhibitory control Control > ADHD 0·040 0·052
15 27 Attention – 0·447 0·847
18 23 Other cognitive domains – 0·440 0·520
C· Stimulus type
Number of
Experiments Stimulus Type Contrasts
P-value
TFCE cFWE
21 65 Neutral – 0·079 0·036
22 41 Neutral Control > ADHD 0·048 0·244
23 24 Neutral ADHD > Control 0·104 0·110
rs-fMRI: resting-state functional magnetic resonance imaging; task-fMRI: task-based functional magnetic resonance imaging; VBM: voxel-based morphometry; TFCE: threshold-free cluster enhancement; cFWE: cluster-level family-wise error.
ACCEPTED MANUSCRIP
T
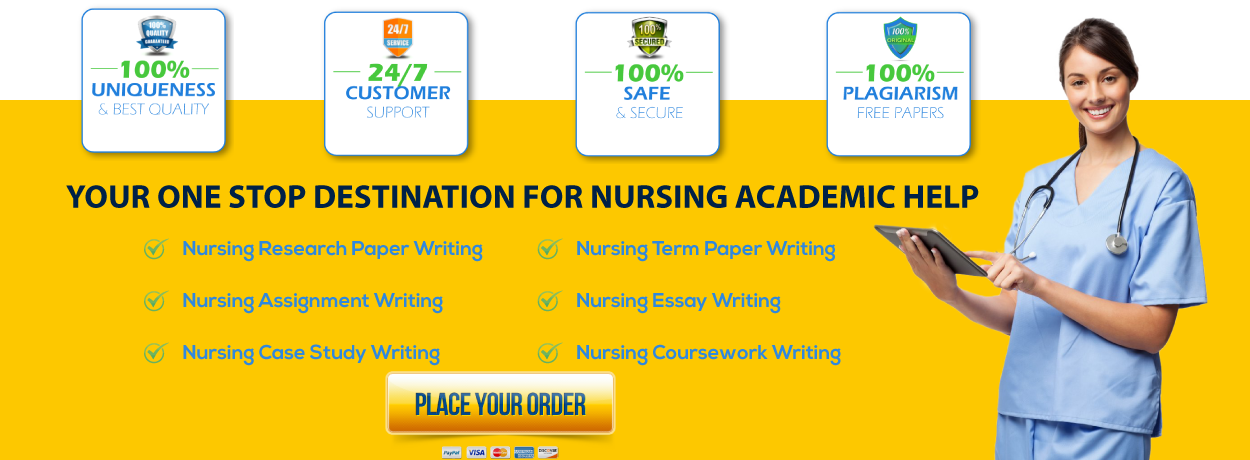