LETTERdoi:10.1038/nature13408
Altitude adaptation in Tibetans caused byintrogression of Denisovan-like DNAEmilia Huerta-Sánchez1,2,3*, Xin Jin1,4*, Asan1,5,6*, Zhuoma Bianba7*, Benjamin M. Peter2, Nicolas Vinckenbosch2, Yu Liang1,5,6,Xin Yi1,5,6, Mingze He1,8, Mehmet Somel9, Peixiang Ni1, Bo Wang1, Xiaohua Ou1, Huasang1, Jiangbai Luosang1, Zha Xi Ping Cuo10,Kui Li11, Guoyi Gao12, Ye Yin1, Wei Wang1, Xiuqing Zhang1,13,14, Xun Xu1, Huanming Yang1,15,16, Yingrui Li1, Jian Wang1,16,Jun Wang1,15,17,18,19 & Rasmus Nielsen1,2,20,21
As modern humans migrated out of Africa, they encountered manynew environmental conditions, including greater temperature extremes,different pathogens and higher altitudes. These diverse environmentsare likely to have acted as agents of natural selection and to have led tolocal adaptations. One of the most celebrated examples in humans isthe adaptation of Tibetans to the hypoxic environment of the high-altitude Tibetan plateau1–3. A hypoxia pathway gene, EPAS1, was pre-viously identified as having the most extreme signature of positiveselection in Tibetans4–10, and was shown to be associated with differ-ences in haemoglobin concentration at high altitude. Re-sequencingthe region around EPAS1 in 40 Tibetan and 40 Han individuals, wefind that this gene has a highly unusual haplotype structure that canonly be convincingly explained by introgression of DNA from Deni-sovan or Denisovan-related individuals into humans. Scanning alarger set of worldwide populations, we find that the selected haplo-type is only found in Denisovans and in Tibetans, and at very lowfrequency among Han Chinese. Furthermore, the length of the hap-lotype, and the fact that it is not found in any other populations, makesit unlikely that the haplotype sharing between Tibetans and Deni-sovans was caused by incomplete ancestral lineage sorting ratherthan introgression. Our findings illustrate that admixture with otherhominin species has provided genetic variation that helped humansto adapt to new environments.
The Tibetan plateau (at greater than 4,000 m) is inhospitable to humansettlement because of low atmospheric oxygen pressure (,40% lowerthan at sea level), cold climate and limited resources (for example, sparsevegetation). Despite these extreme conditions, Tibetans have success-fully settled in the plateau, in part due to adaptations that confer lowerinfant mortality and higher fertility than acclimated women of low-altitude origin. The latter tend to have difficulty bearing children at highaltitude, and their offspring typically have low birth weights compared tooffspring from women of high-altitude ancestry1,2. One well-documentedpregnancy-related complication due to high altitude is the higher inci-dence of preeclampsia2,11 (hypertension during pregnancy). In addition,the physiological response to low oxygen differs between Tibetans andindividuals of low-altitude origin. For most individuals, acclimatiza-tion to low oxygen involves an increase in blood haemoglobin levels.However, in Tibetans, the increase in haemoglobin levels is limited3,presumably because high haemoglobin concentrations are associatedwith increased blood viscosity and increased risk of cardiac events, thusresulting in a net reduction in fitness12,13.
Recently, the genetic basis underlying adaptation to high altitude inTibetans was elucidated4–10 using exome and single nucleotide polymor-phism (SNP) array data. Several genes seem to be involved in the res-ponse but most studies identified EPAS1, a transcription factor inducedunder hypoxic conditions, as the gene with the strongest signal of Tibetanspecific selection4–10. Furthermore, SNP variants in EPAS1 showed sig-nificant associations with haemoglobin levels in the expected directionin several of these studies; individuals carrying the derived allele havelower haemoglobin levels than individuals homozygous for the ances-tral allele. Here, we re-sequence the complete EPAS1 gene in 40 Tibetanand 40 Han individuals at more than 2003 coverage to further char-acterize this impressive example of human adaptation. Remarkably, wefind the source of adaptation was likely to be due to the introduction ofgenetic variants from archaic Denisovan-like individuals (individualsclosely related to the Denisovan individual from the Altai Mountains14)into the ancestral Tibetan gene pool.
After applying standard next-generation sequencing filters (see Meth-ods), we call a total of 477 SNPs in a region of approximately 129 kilo-bases (kb) in the combined Han and Tibetan samples (SupplementaryTables 1 and 2). We compute the fixation index (FST; see Methods) betweenHan and Tibetans, and confirm that it is highly elevated in the EPAS1region as expected under strong local selection (Extended Data Fig. 1).Indeed, by comparison to 26 populations from the Human GenomeDiversity Panel15,16 (Fig. 1) it is clear that the variants in this region arefar more differentiated than one would expect given the average genome-wide differentiation between Han and Tibetans (FST ,0.02, ref. 4). Theonly other genes with comparably large frequency differences betweenany closely related populations are the previously identified loci associ-ated with lighter skin pigmentation in Europeans, SLCA45A2 and HERC2(refs 17–20), although in these examples the populations compared (forexample, Hazara and French, Brahui and Russians) are more geneticallydifferentiated than Han and Tibetans. In populations as closely relatedas Han and Tibetans, we find no examples of SNPs with as much differen-tiation as seen in EPAS1, illustrating the uniqueness of its selection signal.
FST is particularly elevated in a 32.7-kb region containing the 32 mostdifferentiated SNPs (green box in Extended Data Fig. 1 and Supplemen-tary Table 3), which is the best candidate region for the advantageousmutation(s). We therefore focus the subsequent analyses primarily onthis region. Phasing the data (see Methods) to identify Han and Tibetanhaplotypes in this region (Fig. 2), we find that Tibetans carry a high-frequency haplotype pattern that is strikingly different from both their
*These authors contributed equally to this work.
1BGI-Shenzhen, Shenzhen 518083, China. 2Department of Integrative Biology, University of California, Berkeley, California 94720 USA. 3School of Natural Sciences, University of California, Merced,California 95343 USA. 4School of Bioscience and Bioengineering, South China University of Technology, Guangzhou 510006, China. 5Binhai Genomics Institute, BGI-Tianjin, Tianjin 300308, China.6Tianjin Translational Genomics Center, BGI-Tianjin, Tianjin 300308, China. 7The People’s Hospital of Lhasa, Lhasa 850000, China. 8Bioinformatics and Computational Biology Program, Iowa StateUniversity, Ames, Iowa 50011, USA. 9Department of Biological Sciences, Middle East Technical University, 06800 Ankara, Turkey. 10The Second People’s Hospital of Tibet Autonomous Region, Lhasa850000, China. 11The People’s Hospital of the Tibet Autonomous Region, Lhasa 850000, China. 12The hospital of XiShuangBanNa Dai Nationalities, Autonomous Jinghong, 666100 Yunnan, China. 13TheGuangdong Enterprise Key Laboratory of Human Disease Genomics, BGI-Shenzhen, 518083 Shenzhen, China. 14Shenzhen Key Laboratory of Transomics Biotechnologies, BGI-Shenzhen, 518083Shenzhen, China. 15Princess Al Jawhara Center of Excellence in the Research of Hereditary Disorders, King Abdulaziz University, Jeddah 21589, Saudi Arabia. 16James D. Watson Institute of GenomeScience, 310008 Hangzhou, China. 17Department of Biology, University of Copenhagen, Ole MaaløesVej 5, 2200 Copenhagen, Denmark. 18Macau University of Science and Technology, AvenidaWai long,Taipa, Macau 999078,China. 19Departmentof Medicine, University of Hong Kong 999077,Hong Kong. 20Departmentof Statistics, University of California, Berkeley, California 94720,USA. 21Department ofBiology, University of Copenhagen, 2200 Copenhagen, Denmark.
1 9 4 | N A T U R E | V O L 5 1 2 | 1 4 A U G U S T 2 0 1 4
Macmillan Publishers Limited. All rights reserved©2014
minority haplotypes and the common haplotype observed in Han ChineseFor example, the region harbours a highly differentiated 5-SNP haplo-type motif (AGGAA) within a 2.5-kb window that is only seen in Tibetansamples and in none of the Han samples (the first five SNPs in Sup-plementary Table 3, and blue arrows in Fig. 2). The pattern of geneticvariation within Tibetans appears even more unusual because none ofthe variants in the five-SNP motif is present in any of the minority hap-lotypes of Tibetans. Even when subject to a selective sweep, we wouldnot generally expect a single haplotype to contain so many unique muta-tions not found on other haplotypes.
We investigate whether a model of selection on either a de novo muta-tion (SDN) or selection on standing variation (SSV) could possibly leadto so many fixed differences between haplotype classes in such a shortregion within a single population. To do so, we simulate a 32.7-kb regionunder these models assuming different strengths of selection and con-ditioning on the current allele frequency in the sample (see Methods).We find that the observed number of fixed differences between the hap-lotype classes is significantly higher than what is expected by simula-tions under any of the models explored (Extended Data Fig. 2). Thusthe degree of differentiation between haplotypes is significantly largerthan expected from mutation, genetic drift and directional selection alone.In other words, it is unlikely (P , 0.02 under either a SSV scenario orunder a SDN scenario) that the high degree of haplotype differentiationcould be caused by a single beneficial mutation landing by chance on abackground of rare SNPs, which are then brought to high frequency byselection. The remaining explanations are the presence of strong epistasisbetween many mutations, or that a divergent population introduced thehaplotype into Tibetans by gene flow or through ancestral lineage sorting.
We search for potential donor populations in two different data sets:the 1000 Genomes Project21 and whole genome data from ref. 14. Weoriginally defined the EPAS1 32.7-kb region boundaries by the level ofobserved differentiation between the Tibetans and Han only (Supplemen-tary Table 3, Extended Data Fig. 1 and Fig. 2) as described in the pre-vious section. In that region, the most common haplotype in Tibetansis tagged by the distinctive five-SNP motif (AGGAA; the first five SNPsin Fig. 2), not found in any of our 40 Han samples. We first focus on thisfive-SNP motif and determine whether it is unique to Tibetans or if it isfound in other populations.
Intriguingly, when we examine the 1000 Genomes Project data set, wediscover that the Tibetan five-SNP motif (AGGAA) is not present in any
of these populations, except for a single CHS (Southern Han Chinese)and a single CHB (Beijing Han Chinese) individual. Extended Data Fig. 3contains the frequencies of all the haplotypes present in the fourteen1000 Genomes populations21 at these five SNP positions. Furthermore,when we examine the data set from ref. 14 containing both modern (Pap-uan, San, Yoruba, Mandeka, Mbuti, French, Sardinian, Han Dai, Dinka,Karitiana, and Utah residents of northern and western European ances-try (CEU)) and archaic (high-coverage Denisovan and low-coverageCroatian Neanderthal) human genomes14, we discover that the five-SNPmotif is completely absent in all of their modern human population sam-ples (Supplementary Table 4). Therefore, apart from one CHS and oneCHB individual, none of the other extant human populations sampledto date carry this five-SNP haplotype. Notably, the Denisovan haplo-type at these five sites (AGGAA) exactly matches the five-SNP Tibetanmotif (Supplementary Table 4 and Extended Data Fig. 3).
We observe the same pattern when focusing on the entire 32.7-kbregion and not just the five-SNP motif. Twenty SNPs in this region haveunusually high frequency differences of at least 0.65 between Tibetansand all the other populations from the 1000 Genomes Project (ExtendedData Fig. 4). However, in Tibetans, 15 out of these 20 SNPs are identicalto the Denisovan haplotype generating an overall pattern of high hap-lotype similarity between the selected Tibetan haplotype and the Deni-sovan haplotype (Supplementary Tables 5–7). Interestingly, five of theseSNPs in the region are private SNPs shared between Tibetans and theDenisovan, but not shared with any other population worldwide, except
Genome-wide FST
Max
imum
freq
uenc
y di
ffere
nce
0.00 0.05 0.10 0.15 0.200.4
0.5
0.6
0.7
0.8
0.9
1.0
EPAS1
SLC45A2
SLC45A2SLC45A2
HERC2
Figure 1 | Genome-wide FST versus maximal allele frequency difference.The relationship between genome-wide FST (x axis) computed for each pair ofthe 26 populations and maximal allele frequency difference (y axis), firstexplored in ref. 19. Maximal allele frequency difference is defined as the largestfrequency difference observed for any SNP between a population pair. The 26populations are from the Human Genome Diversity Panel (HGDP). Thelabels highlight genes that harbour SNPs previously identified as having stronglocal adaptation. The grey points represent the observed relationship betweenpopulation differentiation (FST ) and maximal allele frequency difference;the more differentiated populations tend to have mutations with largerfrequency differences. The star symbol and the yellow symbols representoutliers; these are populations that are not highly differentiated but where wefind some mutations that have higher frequency differences than expected(light blue line).
Tibe
tans
Han
Chi
nese
***** ** * * * * ** * ** * * * * * * * *** ** * **
Figure 2 | Haplotype pattern in a region defined by SNPs that are at highfrequency in Tibetans and at low frequency in Han Chinese. Each column isa polymorphic genomic location (95 in total), each row is a phased haplotype(80 Han and 80 Tibetan haplotypes), and the coloured column on the leftdenotes the population identity of the individuals. Haplotypes of the Denisovanindividual are shown in the top two rows (green). The black cells represent thepresence of the derived allele and the grey space represents the presence ofthe ancestral allele (see Methods). The first and last columns correspond to thefirst and last positions in Supplementary Table 3, respectively. The red andblue arrows indicate the 32 sites in Supplementary Table 3. The blue arrowsrepresent a five-SNP haplotype block defined by the first five SNPs in the32.7-kb region. Asterisks indicate sites at which Tibetans share a derived allelewith the Denisovan individual.
LETTER RESEARCH
1 4 A U G U S T 2 0 1 4 | V O L 5 1 2 | N A T U R E | 1 9 5
Macmillan Publishers Limited. All rights reserved©2014
for two SNPs at low frequency in Han Chinese (Extended Data Fig. 4and Supplementary Table 7).
If we consider all SNPs (not just the most differentiated) in the 32.7-kbregion annotated in humans, to build a haplotype network22 using the40 most common haplotypes, we observe a clear pattern in which theTibetan haplotype is much closer to the Denisovan haplotype than anymodern human haplotype (Fig. 3 and Extended Fig. 5a; see ExtendedData Fig. 6a, b for haplotype networks constructed using other criteria).Furthermore, we find that the Tibetan haplotype is slightly more diver-gent from other modern human populations than the Denisovan haplo-type is, a pattern expected under introgression (see Methods and ExtendedData Fig. 5b). Raw sequence divergence for all sites and all haplotypesshows a similar pattern (Extended Data Fig. 7). Moreover, the divergencebetween the common Tibetan haplotype and Han haplotypes is largerthan expected for comparisons among modern humans, but well withinthe distribution expected from human–Denisovan comparisons (ExtendedData Fig. 8). Notably, sequence divergence between the Tibetans’ mostcommon haplotype and Denisovan is significantly lower (P 5 0.0028)than expected from human–Denisovan comparisons (Extended DataFig. 8). We also find that the number of pairwise differences betweenthe common Tibetan haplotype and the Denisovan haplotype (n 5 12)is compatible with the levels one would expect from mutation accu-mulation since the introgression event (see Methods for Extended DataFig. 8). Finally, if we compute D (ref. 14) and S* (refs 23, 24), two statis-tics that have been designed to detect archaic introgression into mod-ern humans, we obtain significant values (D-statistic P , 0.001, and S*P # 0.035) for the 32.7-kb region using multiple null models of no geneflow (see Methods, Supplementary Tables 8–10, and Extended DataFigs 9 and 10a).
Thus, we conclude that the haplotype associated with altitude adapta-tion in Tibetans is likely to be a product of introgression from Denisovanor Denisovan-related populations. The only other possible explanation
is ancestral lineage sorting. However, this explanation is very unlikelyas it cannot explain the significant D and S* values and because it wouldrequire a long haplotype to be maintained without recombination sincethe time of divergence between Denisovans and humans (estimated tobe at least 200,000 years (ref. 14)). The chance of maintaining a 32.7-kbfragment in both lineages throughout 200,000 years is conservativelyestimated at P 5 0.0012 assuming a constant recombination of 2.3 31028 per base pair (bp) per generation (see Methods). Furthermore, thehaplotype would have to have been independently lost in all African andnon-African populations, except for Tibetans and Han Chinese.
We have re-sequenced the EPAS1 region and found that Tibetans har-bour a highly differentiated haplotype that is only found at very low fre-quency in the Han population among all the 1000 Genomes populations,and is otherwise only observed in a previously sequenced Denisovanindividual14. As the haplotype is observed in a single individual in bothCHS and CHB samples, it suggests that it was introduced into humansbefore the separation of Han and Tibetan populations, but subject toselection in Tibetans after the Tibetan plateau was colonized. Alterna-tively, recent admixture from Tibetans to Hans may have introducedthe haplotype to nearby Han populations outside Tibet. The CHS andCHB individuals carrying the five-SNP Tibetan–Denisovan haplotype(Extended Data Fig. 3) show no evidence of being recent migrants fromTibet (see Methods and Extended Data Fig. 10b), suggesting that if thehaplotype was carried from Tibet to China by migrants, this migrationdid not occur within the last few generations.
Previous studies examining the genetic contributions of Denisovansto modern humans14,25 suggest that Melanesians have a much larger Deni-sovan component than either Han or Mongolians, even though the latterpopulations are geographically much closer to the Altai mountains14,25.Interestingly, the putatively beneficial Denisovan EPAS1 haplotype isnot observed in modern-day Melanesians or in the high-coverage AltaiNeanderthal26 (Supplementary Table 4). Evidence has been found for
I
II
III
IV
V
VI
VII
VIII
IXX
XI
XII
XIII
XIV
XV
XVI
XVII
XVIIIXIX
XX
XXI
XXII
XXIII
XXIV
XXV
XXVI
XXVII
XXVIII
XXIX
XXX
XXXI
XXXII
XXXIII
XXXIV
XXXV
XXXVI
XXXVIIXXXVIII
XXXIX
XL
XLI
CEU
YRICHB
CHS
GBR
PUR
JPT
LWK
ASWTibetan
Denisovan TSI
FIN
MXL
CLM
2 10 20
35
9
140
Figure 3 | A haplotype network based on the number of pairwise differencesbetween the 40 most common haplotypes. The haplotypes were defined fromall the SNPs present in the combined 1000 Genomes and Tibetan samples:515 SNPs in total within the 32.7-kb EPAS1 region. The Denisovan haplotypeswere added to the set of the common haplotypes. The R software packagepegas23 was used to generate the figure, using pairwise differences as distances.Each pie chart represents one unique haplotype, labelled with Roman numerals,and the radius of the pie chart is proportional to the log2(number ofchromosomes with that haplotype) plus a minimum size so that it is easierto see the Denisovan haplotype. The sections in the pie provide the breakdownof the haplotype representation amongst populations. The width of the edgesis proportional to the number of pairwise differences between the joinedhaplotypes; the thinnest edge represents a difference of one mutation. The
legend shows all the possible haplotypes among these populations. Thenumbers (1, 9, 35 and 40) next to an edge (the line connecting two haplotypes)in the bottom right are the number of pairwise differences between thecorresponding haplotypes. We added an edge afterwards between the Tibetanhaplotype XXXIII and its closest non-Denisovan haplotype (XXI) to indicate itsdivergence from the other modern human groups. Extended Data Fig. 5acontains all the pairwise differences between the haplotypes presented in thisfigure. ASW, African Americans from the south western United States; CEU,Utah residents with northern and western European ancestry; GBR, British;FIN, Finnish; JPT, Japanese; LWK, Luhya; CHS, southern Han Chinese; CHB,Han Chinese from Beijing; MXL, Mexican; PUR, Puerto Rican; CLM,Colombian; TSI, Toscani; YRI, Yoruban. Where there is only one line within apie chart, this indicates that only one population contains the haplotype.
RESEARCH LETTER
1 9 6 | N A T U R E | V O L 5 1 2 | 1 4 A U G U S T 2 0 1 4
Macmillan Publishers Limited. All rights reserved©2014
Denisovan admixture throughout southeast Asia (as well as in Melane-sians) based on a global analysis of SNP array data from 1,600 individualsfrom a diverse set of populations27, and this finding has been recentlyconfirmed by ref. 26. Therefore, it appears that sufficient archaic admix-ture into populations near the Tibetan region occurred to explain thepresence of this Denisovan haplotype outside Melanesia. Furthermore,the haplotype may have been maintained in some human populations,including Tibetans and their ancestors, through the action of naturalselection.
Recently, a few studies have supported the idea of adaptive introgres-sion from archaic humans to modern humans as having a role in theevolution of immunity-related genes (HLA (ref. 28) and STAT2 (ref. 29))and in the evolution of skin pigmentation genes (BNC2 (refs 23, 30)).Our findings imply that one of the most clear-cut examples of humanadaptation is likely to be due to a similar mechanism of gene flow fromarchaic hominins into modern humans. With our increased understand-ing that human evolution has involved a substantial amount of geneflow from various archaic species, we are now also starting to under-stand that adaptation to local environments may have been facilitatedby gene flow from other hominins that may already have been adaptedto those environments.
METHODS SUMMARYDNA samples included in this work were extracted from peripheral blood of 41unrelated Tibetan individuals living at more than 4,300 m above sea level withinthe Himalayan Plateau, with informed consent. Tibetan identity was based on self-reported family ancestry. The individuals were from two villages of Dingri (4,300 maltitude) and Naqu (4,600 m altitude). These individuals are a subset of the 50 indi-viduals exome-sequenced analysed in ref. 4. Samples of 40 Han Chinese (CHB) arefrom the 1000 Genomes Project. A combined strategy of long-range PCR and next-generation sequencing was used to decipher the whole EPAS1 gene and its 630-kbflanking region. We designed 38 pairs of long-range PCR primers to amplify theregion in 41 Tibetan and 40 Han individuals. PCR products from all individualswere fragmented and indexed, then sequenced to higher than 260-fold depth foreach individual with the Illumina Hiseq2000 sequencer. The reads were aligned tothe UCSC human reference genome (hg18) using the SOAPaligner. Genotypes ofeach individual at every genomic location of the EPAS1 gene and the flanking regionwere called by SOAPsnp. To make comparisons with the 40 Han easier, we only used40 Tibetan samples for this study.
Online Content Methods, along with any additional Extended Data display itemsandSourceData, are available in the online version of the paper; references uniqueto these sections appear only in the online paper.
Received 17 December 2013; accepted 28 April 2014.Published online 2 July; corrected online 13 August 2014 (see full-text HTMLversion for details).
1. Moore, L. G., Young, D., McCullough, R. E., Droma, T. & Zamudio, S. Tibetanprotection from intrauterine growth restriction (IUGR) and reproductive loss athigh altitude. Am. J. Hum. Biol. 13, 635–644 (2001).
2. Niermeyer, S. et al. Child health and living at high altitude. Arch. Dis. Child. 94,806–811 (2009).
3. Wu, T. et al. Hemoglobin levels in Quinghai-Tibet: different effects of gender forTibetans vs. Han. J. Appl. Physiol. 98, 598–604 (2005).
4. Yi, X. et al. Sequencing of 50 human exomes reveals adaptation to high altitude.Science 329, 75–78 (2010).
5. Bigham, A. et al. Identifying signature of natural selection in Tibetan and Andeanpopulations using dense genome scan data. PLoS Genet. 6, e1001116 (2010).
6. Simonson, T.S.et al.Geneticevidence forhigh-altitudeadaptation inTibet.Science329, 72–75 (2010).
7. Beall, C. M. et al. Natural selection on EPAS1 (HIF2a) associated with lowhemoglobin concentration in Tibetan highlanders. Proc. Natl Acad. Sci. USA 107,11459–11464 (2010).
8. Peng, Y. et al. Genetic variations in Tibetan populations and high-altitudeadaptation at the Himalayas. Mol. Biol. Evol. 28, 1075–1081 (2011).
9. Xu, S. et al. A genome-wide search for signals of high-altitude adaptation inTibetans. Mol. Biol. Evol. 28, 1003–1011 (2011).
10. Wang, B. et al. On the origin of Tibetans and their genetic basis in adaptinghigh-altitude environments. PLoS ONE 6, e17002 (2011).
11. Moore, L. G. et al. Maternal adaptation to high-altitude pregnancy: an experimentof nature—a review. Placenta 25, S60–S71 (2004).
12. Vargas, E. & Spielvogel, H. Chronic mountain sickness, optimal hemoglobin, andheart disease. High Alt. Med. Biol. 7, 138–149 (2006).
13. Yip, R. Significance of anabnormally low orhighhemoglobin concentrationduringpregnancy: special consideration of iron nutrition1’2’3. Am. J. Clin. Nutr. 72,272S–279S (2000).
14. Meyer, M. et al. A high-coverage genome sequence from an archaic Denisovanindividual. Science 338, 222–226 (2012).
15. Li, J. Z. et al. Worldwide human relationships inferred from genome-wide patternsof variation. Science 319, 1100–1104 (2008).
16. Rosenberg, N. A. Standardized subsets of the HGDP-CEPH Human GenomeDiversityCell LinePanel, accounting for atypical andduplicatedsamples andpairsof close relatives. Ann. Hum. Genet. 70, 841–847 (2006).
17. Soejima, M. & Koda, Y. Population differences of two coding SNPs. inpigmentation-related genes SLC24A5 and SLC45A2. Int. J. Legal Med. 121, 36–39(2007).
18. Sulem, P. et al. Genetic determinants of hair, eye and skin pigmentation inEuropeans. Nature Genet. 39, 1443–1452 (2007).
19. Coop, G. et al. The role of geography in human adaptation. PLoS Genet. 5,e1000500 (2009).
20. Pickrell, J. K. et al. Signals of recent positive selection in a worldwide sample ofhuman populations. Genome Res. 19, 826–837 (2009).
21. An integrated map of genetic variation from 1,092 human genomes. Nature 491,56–65 (2012).
22. Paradis, E. Pegas: an R package for population genetics with an integrated–modular approach. Bioinformatics 26, 419–420 (2010).
23. Vernot, B. & Akey, J. Resurrecting Surviving neandertal lineages from modernhuman genomes. Science (2014).
24. Plagnol, V. & Wall, J. D. Possible ancestral structure in human populations. PLoSGenet. 2, e105 (2006).
25. Reich, D. et al. Genetic history of an archaic hominin group from Denisova cave inSiberia. Nature 468, 1053–1060 (2010).
26. Prüfer, K. et al. The complete genome sequence of a Neanderthal from the AltaiMountains. Nature 505, 43–49 (2014).
27. Skoglund, P.& Jakobsson, M.Archaic human ancestry inEast Asia. Proc. Natl Acad.Sci. USA 108, 18301–18306 (2011).
28. Abi-Rached, L. et al. The shaping of modern human immune systems bymultiregional admixture with archaic humans. Science 334, 89–94 (2011).
29. Mendez, F. L., Watkins, J. C. & Hammer, M. F. A haplotype at STAT2 introgressedfrom Neanderthals and serves as a candidate of positive selection in Papua NewGuinea. Am. J. Hum. Genet. 91, 265–274 (2012).
30. Sankararaman, S. et al. The genomic landscape of Neanderthal ancestry inpresent-day humans. Nature (2014).
Supplementary Information is available in the online version of the paper.
Acknowledgements This researchwas fundedby the StateKey Development Programfor Basic Research of China, 973 Program (2011CB809203, 2012CB518201,2011CB809201, 2011CB809202), China National GeneBank-Shenzhen andShenzhen Key Laboratory of Transomics Biotechnologies (no. CXB201108250096A).This work was also supported by research grants from the US NIH; R01HG003229 toR.N. and R01HG003229-08S2 to E.H.S. We thank F. Jay, M. Liang and F. Casey foruseful discussions.
Author Contributions R.N., Ji.W. and Ju.W. supervised the project. X.J., A., Z.B., Y.L., X.Y.,M.H., P.N., B.W., X.O., H., J.L., Z.X.P.C., K.L., G.G., Y.Y., W.W., X.Z., X.X., H.Y., Y.L., Ji.W. andJu.W. collected and generated the data, and performed the preliminary bioinformaticanalyses to call SNPs and indels from the raw data. E.H.-S. andN.V. filtered the data andB.M.P. phased the data. E.H.-S. performed the majority of the population geneticanalysis with some contributions from B.M.P. and M.S. E.H.-S. and R.N. wrote themanuscript with critical input from all the authors.
Author Information Sequencedatahavebeendeposited in theSequenceReadArchiveunder accession number SRP041218. Reprints and permissions information isavailable at www.nature.com/reprints. The authors declare no competing financialinterests. Readers are welcome to comment on the online version of the paper.Correspondence and requests for materials should be addressed toJu.W. (wangj@genomics.cn) or Ji.W. (wangjian@genomics.cn) orR.N. (rasmus_nielsen@berkeley.edu).
LETTER RESEARCH
1 4 A U G U S T 2 0 1 4 | V O L 5 1 2 | N A T U R E | 1 9 7
Macmillan Publishers Limited. All rights reserved©2014
METHODSDNA samples. DNA samples included in this work were extracted from peripheralblood of 41 unrelated Tibetan individuals living at more than 4,300 m above sea levelwithin the Himalayan Plateau. Tibetan identity was based on self-reported familyancestry. The individuals were from two villages of Dingri (20 samples prefixed DR(Dingri); 4300m altitude) and Naqu (21 samples prefixed NQ (Naqu); 4,600 maltitude). All participants gave a self-report of at least three generations living at thesampling site, and provided informed consent for this study. These individuals area subset of the 50 individuals exome-sequenced from ref. 4. Samples of Han Chinese(CHB) are from the 1000 Genomes Project21 (40 samples prefixed NA in the 1000Genomes Project).
A combined strategy of long-range PCR and next-generation sequencing wasused to decipher the whole EPAS1 gene and its 630-kb flanking region (147 kb intotal). We designed 38 pairs of long-range PCR primers to amplify the region in 41Tibetan and 40 Han individuals. PCR products from all individuals were fragmen-ted and indexed, then sequenced to higher than 260-fold depth for each individualwith the Illumina Hiseq2000 sequencer. The reads were aligned to the UCSC humanreference genome (hg18) using the SOAPaligner31. Genotypes of each individual atevery genomic location of the EPAS1 gene and the flanking region were called bySOAPsnp32. To make comparisons easier with the Han samples, we only used 40Tibetan samples for this study.Data filtering. The coverage for each individual is roughly 2003. Genotypes ofeach individual at every site in the EPAS1 gene and the flanking region were calledby SOAPsnp32, which resulted in 700 SNPs in the combined Tibetan–Han sample.However, we filtered out some sites post genotype calling by performing standardfilters that are applied in the analyses of next generation sequencing data. Specifi-cally, of the 700 SNPs called, we removed SNPs that fell into the following categories:SNPs that were not in Hardy–Weinberg equilibrium; SNPs that were located inhard-to-map regions (the SNP is located at a position with mappability 5 0, usingthe Duke Unique 35 track); SNPs where more than half the heterozygote indivi-duals have a statistically unequal distribution of counts for the two alleles (that is,the counts of the two alleles deviate from the expectation of 50% of counts for eachassuming a binomial distribution); SNPs with different quality-score distributionsfor the two alleles; SNPs that fell on or near a deletion or insertion; and SNPs thatwere tri-allelic. A total of 477 SNPs in the combined sample remained after apply-ing all the filters. Within Tibetans, 354 sites (out of the 477 sites) were SNPs, andwithin Han Chinese, 364 sites (out of the 477) were SNPs. After filtering, we usedBeagle to phase the Tibetan and Han individuals together33.Human Genome Diversity Panel data. We downloaded the HGDP SNP datafrom the University of Chicago website (http://hgdp.uchicago.edu/data/plink_data/)and followed the filtering criteria in ref. 34. We used the formula of ref. 35 to com-pute FST between pairs of populations. We used the intersection of SNPs betweenthe 50 Tibetan exomes from ref. 7 and the HGDP SNPs, resulting in 8,362 SNPs.Note, the number 354 quoted in the previous section refers to Tibetan SNPs fromthe full re-sequencing of the EPAS1 gene in this study.
We calculated FST for each pair of populations and also scored the frequencies ofthe SNP with the largest frequency difference between pairs of populations. Usingthe genotypes from the 26 populations we have re-created Fig. 2a of ref. 34 using theSNPs overlapping in two data sets: the 50 Tibetan exomes data set and the HGDP15,16
data set. The re-created figure (Fig. 1) displays the maximum SNP frequency differ-ence against the mean FST across all SNPs for each pair of the HGDP populations.We added one data point to this figure consisting of the mean Fst between Tibetansand Han (FST is approximately 0.018) and the SNP with the largest frequency dif-ference between Han Chinese and Tibetans (approximately 0.8), which is a SNP inthe EPAS1 gene.Tibetan and Han haplotypes at the 32.7-kb highly differentiated region. The32.7-kb region was identified as the region of highest genetic differentiation betweenTibetans and Han Chinese (green box in Extended Data Fig. 1), providing the stron-gest candidate region for the location of the selective sweep. To examine the haplo-types in this region, we first filtered out SNPs that occurred with a frequency of #5%or $95% in both the Tibetan and Han samples; that is, SNPs that were very commonor very rare in both populations simultaneously. We computed the number ofpairwise differences between every pair of haplotypes. We then ordered the hap-lotypes based on their number of pairwise distances from the most common hap-lotype in each population separately. Figure 2 is generated using the heatmap.2function from the gplots package of the statistical computing platform R (ref. 36),with derived and ancestral alleles coloured black and light grey, respectively. Weused the chimpanzee sequence to define the ancestral state. However, the chim-panzee allele was missing at one of the 32 most differentiated sites (see arrows inFig. 2), so in that case we used the orangutan and rhesus macaque alleles to definethe ancestral allele.Simulations, selection on a de novo mutation and on standing variation. We usedmsms37 to simulate data for a population of constant size with mutation, recombination
and positive selection affecting a single site. We simulated under conditions of acurrent allele frequency of 69 out of 80 in the population, the observed value in thereal EPAS1 data, so that the beneficial mutation had frequency at the present time.We estimated a Tibetan effective population size of N 5 7,000 (see SupplementaryInformation; section on estimating the effective population size). In addition, weassumed three different selection parameters (2Ns 5 200, 500, 1,000, where s is theselection coefficient of the beneficial mutation) and a recombination rate per bpper generation of 2.3 3 1028 (this is the average recombination rate in the EPAS1gene using the fine-scale estimates from the map of ref. 38). This recombinationestimate is very similar to the estimate from the African American map39 for theEPAS1 gene which is 2.4 3 1028. We set the mutation rate to 2.0 3 1028 per bp pergeneration because this is what we estimated using the patterns of genetic diversityin the EPAS1 gene in Tibetans under an approximate bayesian computation (ABC)framework (see Supplementary Information for details; section on estimating themutation rate). This mutation-rate estimate is similar to the phylogenetic estimatesreviewed in ref. 40. We note that the human–chimpanzee differences in other intro-nic regions in the genome of the same size has a mean (417) and median (410) closeto that found for the EPAS1 32.7-kb region (see Supplementary Information; sectionon the distribution of human–chimpanzee differences in 32.7-kb regions for details),suggesting that this region does not have an unusual mutation rate. In the simula-tions, we examined a region of 32.7 kb around the selected site and grouped thehaplotypes into two groups: those that carried the beneficial allele and those thatdid not. If k is the number of chromosomes carrying the beneficial mutation, wecounted how many mutations within the 32.7-kb region had frequency bigger orequal to (k/80 – 0.05) in the group that harboured the beneficial allele (that is, fixedor almost fixed in that group), and simultaneously had frequency 0 in the other group.
To simulate data for a sweep from standing variation, we used mbs41 and thesame parameters as in the previous set of simulations, but assuming an initial allelefrequency of the advantageous allele of 1% when selection starts. To be able to com-pare the number of almost fixed sites from these simulations to the observed data,we needed to make a call in the Han–Tibetan data set of what could plausibly be theselected site. The most straightforward choice is the site that has the highest Han–Tibetan differentiation; see the circled SNP in Extended Data Fig. 1 (this site alsohas the largest frequency difference (,0.85) between Tibetans and any of the 1000Genomes populations). Tibetan individuals with the derived mutation at this sitewere defined as carrying the selected haplotype, and the remaining individuals weredefined as not carrying the selected haplotype. Then we performed the same count-ing of ‘almost fixed’ sites between these two groups as was done for the simulations.The simulated distribution of almost fixed differences and the real data are shown inExtended Data Fig. 2 (histograms of almost fixed differences).
For the SDN model under a selection parameter of 2Ns 5 200, 500, 1,000, theP values are 0.004, 0.006 and 0.006, respectively. Under SSV with a selection para-meter of 2Ns 5 200, 500, 1,000, the P values are 0.002, 0.012 and 0.015, respectively.We note that increasing the initial frequency of the selected allele (to 5%) also leadsto a smaller number of fixed differences than what we observe in the real data, therebymaking the simulated SSV scenario similarly unlikely (P values are 0.007, 0.01 and0.006 for 2Ns 5 200, 500, 1,000 respectively). We also note that simulating data with asmaller mutation rate will not result in an increase in the number of fixed differences.Five-SNP motif. We identified the contiguous five-SNP haplotype motif that is mostcommon in the 40 Tibetan samples, but entirely absent in the 40 Han individuals(see the five-SNP haplotype defined by the first five blue arrows in Fig. 2). The fiveSNPs comprising this motif (positioned at 46421420, 46422184, 46422521, 46423274and 46423846), span a 2.5-kb region (46423846–46421420, ,2.5 kb) containingno other SNPs (even when including low- and high-frequency SNPs). The genomicpositions of this five-SNP motif were then examined in the phased 1000 Genomes21
data set to compute the frequency of this five-SNP haplotype in the populationssequenced in the 1000 Genomes project (see Extended Data Fig. 3, and below). Wewill refer here to this five-SNP motif as the ‘core’ Tibetan haplotype.Haplotype frequencies at the five-SNP motif in 1000 Genomes data. For allsamples or populations in the 1000 genomes project21, we interrogated the five sitesin the ‘core’ Tibetan haplotype identified in EPAS1, and counted the frequencies ofeach of the unique haplotypes within each population group of the 1000 genomes.The bar-plot in Extended Data Fig. 3 is a summary of these frequencies within eachpopulation, coloured by the unique haplotype sequences present.Haplotype network. We constructed a haplotype network including Tibetans, Deni-sovans and the 1000 Genomes samples (ASW, African American from south westernUnited States; CLM, Colombian; CEU, Utah Residents with Northern and WesternEuropean ancestry; CHB, Han Chinese from Beijing; CHS, Southern Han Chinese;FIN, Finnish; GBR, British; JPT, Japanese; LWK, Luhya; MXL, Mexican; PUR, PuertoRican; TSI, Toscani; YRI, Yoruban) for the 32.7-kb EPAS1 region in the combined1000 Genomes samples. To limit the number of haplotypes to display, we identi-fied the 40 most common haplotypes. There are a total of 515 SNPs in the 32.7-kbEPAS1 region that pass all quality filters. We used the R (ref. 36) software package
RESEARCH LETTER
Macmillan Publishers Limited. All rights reserved©2014
pegas (ref. 22) to build a tree that connects haplotypes based on pairwise differ-ences (Fig. 3). The Denisovan individual is homozygous at all the 515 sites. Notethat for clarity (to reduce the number of colours needed for the plot) and becausethe small sample of Iberians (18) only contain haplotypes observed in other Euro-pean samples, Fig. 3 does not include the Iberians (IBS). We find that the Denisovanhaplotype is closest to the Tibetan haplotypes. Extended Data Fig. 5a contains allthe pairwise differences between the 41 (40 from modern humans and 1 from Deni-sovan) haplotypes in Fig. 3.
The observed haplotype structure is compatible with the introgression hypothesis.As expected under the introgression hypothesis, the Tibetan haplotype is moredistant to the non-Tibetan haplotypes than the Denisovan haplotype because, afterthe admixture event, the introgressed haplotype accumulated extra mutations. Incontrast, the Denisovan haplotype, being the product of DNA extracted from anancient specimen, did not have time to accumulate a similar number of mutations.This effect is illustrated in Extended Data Fig. 5b. For example, the divergence betweenthe introgressed haplotype (that is, the Tibetan haplotype) and the Yoruban hap-lotype would be larger than between the observed Denisovan haplotype and theYoruban haplotype (see Extended Data Fig. 5b and Supplementary Information;section on Extended Data Fig. 5b).Haplotype network. Figure 3 plots the network of the 40 most common haplotypes.Alternatively, we also used the 20 sites such that the frequency difference betweenTibetans and each of the 14 populations from the 1000 Genomes project21 is at least0.65 (see Extended Data Fig. 4) to construct a haplotype network (Extended DataFig. 6a). The resulting region spanned by these SNPs is the same 32.7-kb region asidentified previously by considering sites that are the most differentiated betweenTibetans and Han (Supplementary Table 3). For more details about Extended DataFig. 6a, b, see Supplementary Information; section on haplotype networks con-structed using other criteria).Denisovan–human number of pairwise differences at the EPAS1 32.7-kbregion. We computed the number of pairwise differences as described in Supplemen-tary Information (section on the number of pairwise differences). We removed allthe Denisovan sites that had a genotype quality smaller than 40 and a mappingquality smaller than 30, using the same thresholds as in the Denisovan paper14.This filtering resulted in the removal of 782 sites (out of 32,746). We also removedanother 27 sites within the 32.7-kb region that did not pass our quality filters inTibetans (see the data filtering section). The total number of SNPs in the com-bined Tibetan, 1000 Genomes and the Denisovan samples is 520. For the 32.7-kbregion in EPAS1, we computed the number of pairwise differences between theDenisovan haplotypes and each of Tibetan haplotypes (red histograms, ExtendedData Fig. 7). We also computed the number of pairwise differences between theDenisovan haplotypes and each of the haplotypes in the 1000 Genomes Project’spopulations (see the blue histograms in Extended Data Fig. 7). Notice that for thiscomparison, we compared every site that passes the quality filters even if the site isnot polymorphic in modern humans. This is in contrast to Fig. 3 where we onlyconsidered the sites that are polymorphic in modern humans. Furthermore, if asite is not polymorphic in our sample, we assumed that all of our samples carry thehuman reference allele. We plot two histograms in each panel of Extended DataFig. 7: the distribution of Tibetan–Denisovan comparison (red histogram) and thedistribution of pairwise differences between the Denisovan haplotype and eachpopulation (blue histogram) from the 1000 Genomes Project (Extended Data Fig. 7).Denisovan–modern human divergence and modern human–modern humandivergence. To compute the genomic distribution of modern human–Denisovanpairwise differences we examined all windows of intronic sequence of size 32.7 kb(using a table from Ensembl with the exon boundaries for all genes) from chromo-somes 1 to 6. Within each 32.7-kb region, we removed all the Denisovan sites thathad a genotype quality smaller than 40 and a mapping quality smaller than 30. Wecomputed divergence by computing all the pairwise differences between a humanhaplotype and the Denisovan haplotypes (see Supplementary Information; sectionon the number of pairwise differences) and dividing by the effective sequence length(that is, all the sites in the 32.7-kb region that passed all the filters; a mapping qualityhigher or equal to 30 and a genotype quality higher or equal to 40). We only kept the32.7-kb regions where at least 20,000 sites passed these quality criteria. The modernhumans used in these comparisons were the first 80 CEU chromosomes, the first 80CHS chromosomes and the first 80 CHB chromosomes from the 1000 Genomesdata. If a site was not polymorphic in modern humans, we assumed that they carriedthe reference allele.
We also computed modern human–modern human divergence at the same intro-nic regions. In this case, we compare modern human populations (CHB versus CHS,CHB versus CEU, CHS versus CEU) by comparing all 80 haplotypes in one groupto all 80 haplotypes in the other group for a total of 3 3 80 3 80 comparisons. Thedistributions of modern human–Denisovan and modern human–modern humanpairwise differences are both plotted in Extended Data Fig. 8. We also display thedistribution of Tibetan–Han pairwise differences in the 32.7-kb region of the EPAS1
locus (80 Tibetan and 80 Han for a total of 6,400 comparisons). Finally, we includethe pairwise differences between the Denisovan and the Tibetans computed as inExtended Data Fig. 7, standardized by the number of sites that passed all quality filters.This number (12/31,937) leads to a sequence divergence of 0.000375 for the mostcommon Tibetan haplotypes, and this is indeed significantly lower (P 5 0.0028)than what is expected under the distribution of human–Denisovan divergence (seeExtended Data Fig. 8). Supplementary Table 11 contains the details regarding the12 differences between the Tibetan and the Denisovan haplotypes.
To address further the issue of whether 12 differences are expected between theDenisovans and Tibetans under the introgression hypothesis, we computed thenumber of mutations theoretically expected for an introgressed region of this size,given published estimates of the age of the sample, and the coalescence time withinDenisovans. We assumed that mutations occur as a Poisson process and used theestimates of split times from ref. 26 between the called introgressed Denisovan hap-lotypes and the Denisovan haplotypes (see page 114 of the Supplementary Infor-mation of ref. 26). Using these estimates, the number of expected mutations betweenthe Denisovan haplotype and our introgressed haplotype (the Tibetans’ most com-mon haplotype) is simply: (2 3 tMRCA 2 age) 3 L 3 mu 5 11.25, where tMRCA isthe time to the most recent common ancestor estimated at 394,000 years (394 kyr),mu 5 0.5 3 1029 per bp per year, L 5 32.7 kb, and age is the age of the Denisovansample, which we conservatively set to 100,000 years. Clearly, the observed value of12 mutations is remarkably close to the expected number (11.25). In fact, we wouldneed to observe 17 or more mutations to be able to reject the introgression hypo-thesis at the 5% significance level. If we use our estimate of the mutation rate in theEPAS1 gene, mu 5 1.0 3 1029 per bp per year (2.0 3 1028 per bp per generation),then the expected number of differences is 22.5. Therefore we conclude the numberof differences we observe are compatible with the previous estimates of introgressedDenisovan versus sampled Denisovan sequence divergence.Probability of 32.7-kb haplotype block from shared ancestral lineage. We cal-culate the probability of a haplotype, of length at least 32.7 kb, shared by modernTibetans and the archaic Denisovan due to incomplete ancestral lineage sorting.Let r be the recombination rate per generation per bp. Let t be the length of thehuman and Denisovan branches since divergence. The expected length of a sharedancestral sequence is 1/(r 3 t). Let this expected length 5 L. Assuming an exponen-tial distribution of admixture tracts, the probability of seeing a shared fragment oflength $ m is exp(2m/L). However, conditional on observing the Denisovan nucle-otide at position j, the expected length is the sum of two exponential random vari-ables with expected lengths L, therefore it follows a Gamma distribution with shapeparameter 2, and rate parameter lambda 5 1/L. Inserting numbers for human branchlength after divergence at a conservative lower estimate of 200 kyr, and the Denisovanbranch of 100 kyr (divergence minus the estimated age of the Denisovan sample,which can be as old as 100 kyr (refs 14, 26)), and assuming a generation time of25 years, we get L 5 1/(2.3 3 1028 3 (300 3 103/25)) 5 3623.18 bp, and the proba-bility of a length of at least m 5 32,700 bp is 1 2 GammaCDF(32700, shape 5 2,rate 5 1/L) 5 0.0012. GammaCDF is the Gamma distribution function and thenumbers within the parenthesis are its arguments. Here the recombination of 2.33 1028 is the average recombination rate in EPAS1 calculated from the estimatesin ref. 14. We should mention, both this divergence estimate for the Denisovan–human split and the age of the Denisovan sample are highly conservative14,25,26, sothe actual probability may be considerably less. Also, the haplotype would have tohave been independently lost in all African and non-African populations, exceptfor Tibetans and Han Chinese.Null distributions of D statistics under models of no gene flow. As anotherapproach to assess the probability of an ancestral lineage having given rise to the32.7-kb haplotype that we observe in Tibetans in the absence of gene flow, wecompared D statistics between human populations under simulations42 of severaldemographic models described in ref. 43. D statistics were calculated according toequation (2) in ref. 44. The two modern human populations used in computing Dstatistics are Tibetans and either CHB, CEU or YRI (see Supplementary Informa-tion for details; section on D statistics under Models of no gene flow). All simulationsresults result in P , 0.001 for all comparisons (see Extended Data Fig. 9, Supplemen-tary Tables 8–10 and Supplementary Information).Genome-wide value of D statistics. D statistics have been used to assess genome-wide levels of archaic introgression in previous studies14,25. To assess whether Tibetanscarry more Denisovan admixture than other populations (CEU or CHB), we used theSNP genotype data from ref. 45 and computed D statistics as in ref. 44: D(chimpanzee,Denisovan, Tibetan and CHB) and D(chimpanzee, Denisovan,Tibetan and CEU).At the genome-wide level, using the D statistic, we found no evidence that there ismore Denisovan admixture in Tibetans than in the Han (D 5 0.000504688). Wealso did not find evidence that there is more Denisovan admixture in Tibetansthan in the Europeans (D 5 0.001898642).Empirical distributions of D statistics for 32.7-kb intronic regions. The EPAS132.7-kb region was chosen due to its positive selection signal, and not based on a
LETTER RESEARCH
Macmillan Publishers Limited. All rights reserved©2014
genome-wide analysis of Denisovan introgression. Therefore, we only performedone test when testing for introgression and did not have to correct P values for mul-tiple testing. We do not have Tibetan whole genome sequence data, but as shown inthe previous section, genotype array data suggests that the level of Denisovan intro-gression between Han and Tibetans is similar. Moreover, Tibetans and Han are closelyrelated populations. Therefore, using Han data as a proxy, we can determine whetherthe observed D values at the EPAS1 region (D(TIB, YRI, DEN, chimpanzee) 520.8818433) is an outlier compared to the distribution of D values at other 32.7-kbintronic regions. Using the empirical distribution of D values across chromosomes1 to 22, substituting the 80 Han chromosomes for our 80 Tibetan chromosomesand computing D(HAN, YRI, DEN, chimpanzee) for each 32.7-kb intronic region,we obtain a P , 0.008. However, as the variance in D depends on the number ofinformative sites, this is probably an overestimate of the true P value. In fact, thereare no other regions with as many informative sites and as extreme a D value as thatobserved for EPAS1. This region is clearly a strong outlier.Null distributions of S* statistics under models of no gene flow. As a finalapproach for eliminating the hypothesis of ancestral lineage sorting, we follow themethods of ref. 23 to compute S* (originally derived by ref. 24). S*was designed toidentify regions of archaic introgression. As in the previous section, we used all thefour models of ref. 43 that do not include gene flow and simulated data to computethe null distributions of S*. Distributions are generated from 1000 simulations, andwithin each simulation we have representation of the 80 Tibetan chromosomes,and 20 Yoruban chromosomes as the outgroup. For each simulated data set we followref. 23 and compute S* on a per-chromosome basis, after sampling at random 20chromosomes from the Tibetan group and removing SNPs that are observed in theYoruban chromosomes. The maximum S* is then recorded. The above process iscarried out for 10 random samplings of 20 Tibetan chromosomes and the max-imum of the 10 is the final recorded S*. The exact same procedure is applied to thesimulated data and the real data of 80 Tibetan chromosomes. Extended Data Fig. 10ashows that under all four models, S* is significantly different from the null distri-bution with all the empirical p-values lying below 0.035. The grey vertical line is theS* value computed for the real data. The P values are 0.035, 0.028, 0.019 and 0.017,respectively, for each model (top to bottom).Principal component analyses using Chinese and Tibetan samples. As onesingle CHB individual carries a haplotype that is very similar to the Denisovan hap-lotype in EPAS1 (Extended Data Fig. 6a, b), we wanted to assess whether this simi-larity might be due to recent gene-flow from Tibetans to CHB (Chinese sampleswere from the 1000 Genomes Project; Tibetan samples were from ref. 45). If thatwere true, then we would expect to observe similarities at other loci. Therefore wecompute the first and second principal components using all of chromosome 2. Forsimplicity, we only used chromosome 2 because it contains the EPAS1 gene and has
a sufficiently high number of SNPs to carry out the PCA analysis. We do not havegenome-wide genotype calls for the 40 Tibetan samples considered in this study.Therefore, as a proxy, we used the Tibetan genotype data from ref. 45 and com-pared their Tibetan samples to the CHB and CHS individuals from 1000 Genomes.Extended Data Fig. 10b shows that all the CHB and the CHS individuals clustertogether and principal component 1 clearly separates Tibetans from CHB and CHSindividuals. Furthermore, the CHB individual with the Denisovan EPAS1 haplo-type (Extended Data Figs 6a, b) clearly clusters with other CHB and CHS indivi-duals and do not show any closer genetic affinity with Tibetans. This suggests thatthe CHB individual with a Denisovan-like haplotype in EPAS1 is not a descendantof a recent immigrant from Tibet.
31. Li, R., Li, Y., Kristiansen, K. & Wang, J. SOAP: short oligonucleotide alignmentprogram. Bioinformatics 24, 713–714 (2008).
32. Li, R. et al. SNP detection for massively parallel whole-genome resequencing.Genome Res. 19, 1124–1132 (2009).
33. Browning, B. L. & Browning, S. R. A fast, powerful method for detecting identity bydescent. Am. J. Hum. Genet. 88, 173–182 (2011).
34. Coop, G. et al. The role of geography in human adaptation. PLoS Genet. 5,e1000500 (2009).
35. Reynolds, J., Weir, B. S. & Cockerham, C. C. Estimation of the coancestrycoefficient: basis for a short-term genetic distance. Genetics 105, 767–779(1983).
36. R Development Core Team R: A language and environment for statisticalcomputing http://www.R-project.org/ (R Foundation for Statistical Computing,2011).
37. Ewing, G. & Hermisson, J. MSMS: a coalescent simulation program includingrecombination, demographic structure, and selection at a single locus.Bioinformatics 26, 2064–2065 (2010).
38. Myers, S. et al. A fine-scale map of recombination rates and hotspots across thehuman genome. Science 310, 321–324 (2005).
39. Hinch, A. G. et al. The landscape of recombination in African Americans. Nature476, 170–175 (2011).
40. Scally, A. & Durbin, R. Revising the human mutation rate: implications forunderstanding human evolution. Nature Rev. Genet. 13, 745–753 (2012).
41. Teshima, K. M. & Innan, H. mbs: modifying Hudson’s ms software to generatesamples ofDNAsequenceswith a biallelic site under selection. BMC Bioinformatics10, 166 (2009).
42. Hudson, R. R. Generating samples under a Wright–Fisher neutral model of geneticvariation. Bioinformatics 18, 337–338 (2002).
43. Sankararaman, S. et al. The date of interbreeding between Neandertals andmodern humans. PLoS Genet. 8, e1002947 (2012).
44. Durand, E. Y. et al. Testing for ancient admixture between closely relatedpopulations. Mol. Biol. Evol. 28, 2239–2252 (2011).
45. Simonson, T.S.et al.Geneticevidence forhigh-altitudeadaptation inTibet.Science329, 72–75 (2010).
RESEARCH LETTER
Macmillan Publishers Limited. All rights reserved©2014
Extended Data Figure 1 | FST calculated for each SNP between Tibetan andHan populations. Each dot represents the FST value for each SNP in EPAS1.The x axis is the physical position in the gene. Positions are based on thehg18 build of the human genome. The green box defines a 32.7-kb region wherewe observe the largest genetic differentiation between Han Chinese and
Tibetans. The first and last positions of this 32.7-kb region correspond tothe first and last position of the SNPs listed in Supplementary Table 3. Forcomparison, in ref. 4 the genome-wide FST between Han and Tibetans is 0.02.The site with the largest frequency difference (and therefore largest FST)is circled.
LETTER RESEARCH
Macmillan Publishers Limited. All rights reserved©2014
Extended Data Figure 2 | Distribution of fixed differences. The left panel isthe distribution of fixed differences between two haplotype groups under ascenario of selection on a de novo mutation (see Methods), and the right panelis the distribution under a scenario of selection on standing variation (seeMethods) for a region of size ,32.7 kb. The initial frequency of the selected
allele in the SSV model is 1%. Each row of panels corresponds to differentselection strengths (2Ns) from 200 to 1,000. The red lines mark the number offixed differences observed between the two haplotype classes in the real data forthe given window size.
RESEARCH LETTER
Macmillan Publishers Limited. All rights reserved©2014
Extended Data Figure 3 | Haplotype frequencies for Tibetans, our Hansamples and the populations from the 1000 genomes project for thefive-SNP motif in the EPAS1 region. The y axis is the haplotype frequency.The legend shows all the possible haplotypes for the region considered amongthese populations: ASW, African American from the south western United
States; CEU, Utah Residents with Northern and Western European ancestry;CHB, Han Chinese from Beijing; CHS, Southern Han Chinese; CLM,Colombian; FIN, Finnish; GBR, British; HAN, Han Chinese from Beijing; IBS,Iberian; JPT, Japanese; MXL, Mexican; PUR, Puerto Rican; LWK, Luhya; TSI,Toscani; TIB, Tibetan; YRI, Yoruban (see Methods).
LETTER RESEARCH
Macmillan Publishers Limited. All rights reserved©2014
Extended Data Figure 4 | Derived allele frequency of the SNPs with thelargest frequency difference between Tibetans and the 1000 GenomesProject populations. At these SNPs, the frequency difference between Tibetansand the 1000 Genomes project populations is 0.65 or larger. Positions46571435, 46579689, 46584859 and 46600358 were not called as SNPs in
the 1000 Genomes data, so we assume these positions were fixed for the humanreference allele. Note that even though position 46577251, 46588331, 46594122and 46598025 appear to have a frequency of 0.0 for the populations in the1000 Genomes data, the derived allele in these SNPs are observed at very lowfrequency in at least one population (for example, CHB).
RESEARCH LETTER
Macmillan Publishers Limited. All rights reserved©2014
Extended Data Figure 5 | Differences between haplotypes. a, The full matrixof pairwise differences between all the unique haplotypes in Fig. 3, for the40 most common haplotypes identified in the 1000 Genomes and the Tibetansamples in the 32.7-kb region of EPAS1. The Denisovan haplotype (offrequency two) was added afterwards for comparison. The unique haplotypesare labelled with Roman numerals (here and in Fig. 3), and the Denisovanhaplotype is the first column, haplotype I. Refer to Fig. 3 in the main text and thesupplementary material for the representation of populations for each
haplotype. b, Illustration of the genealogical structure in a model with gene flowfrom Denisovans to Tibet. Letters a–k are the labels for the branch lengths andare adjacent to their corresponding branches. The divergence between modernhuman haplotypes and the introgressed haplotype in Tibetans would be largerthan the haplotypes in other modern human populations and the Denisovanhaplotype (see Methods and Supplementary Information). TIB, CEU and YRIdenote Tibetan, European and Yoruban populations. Note that the lengths iand k are unknown as we do not know when these populations went extinct.
LETTER RESEARCH
Macmillan Publishers Limited. All rights reserved©2014
Extended Data Figure 6 | Other haplotype networks. a, A haplotype networkbased on the number of pairwise differences between 43 unique haplotypesdefined from the 20 most differentiated SNPs between Tibetans and the 14populations from the 1000 Genomes Project. The R software package pegas(ref. 22) was used to generate the figure. The haplotype distances are frompairwise differences. Each pie chart represents one unique haplotype and thesize of the pie chart is proportional to log2(number of chromosomes with thathaplotype). The sections in the pie provide the breakdown of the haplotypesamongst populations. The width of the edges is proportional to the number ofpairwise differences between the joined haplotypes; the thinnest edge widthrepresents a difference of one mutation. The number 57 next to a Tibetanhaplotype is the number of Tibetan chromosomes with that haplotype.
Similarly, the number 1,912 is the number of chromosomes (across severalpopulations) with that haplotype. b, The number of pairwise differencesbetween the Denisovan haplotype and the 43 unique haplotypes defined fromthe 20 most differentiated SNPs between Tibetans and the 14 populations fromthe 1000 Genomes Project (same haplotypes as in a). Each bar is a uniquehaplotype, and they are sorted in increasing order of pairwise differences. Thecolours within each bar represent the proportion of chromosomes with thathaplotype broken down by populations. The numbers on top of each barrepresent the total number of chromosomes within the 1000 Genomes data setand Tibetans that have the haplotype. Note this is the same data set used tocreate the haplotype network in panel a. Supplementary Tables 5 and 6 containthe 43 haplotypes and the frequencies within each of the populations.
RESEARCH LETTER
Macmillan Publishers Limited. All rights reserved©2014
Extended Data Figure 7 | Number of pairwise differences. Red bars are thehistograms of the number of pairwise differences between Denisovan andTibetans. Blue bars are the histograms of the number of pairwise differences
between Denisovan and GBR, CHS, FIN, PUR, CLM, IBS, CEU, YRI, CHB,JPT, LWK, ASW, MXL or TSI. All comparisons are within the 32.7-kb region ofhigh differentiation (green box in Extended Data Fig. 1).
LETTER RESEARCH
Macmillan Publishers Limited. All rights reserved©2014
Extended Data Figure 8 | Divergence distributions. Modern human–Denisovan divergence (see Methods) for intronic regions of size 32.7 kb isplotted in red. Modern human–modern human divergence for the sameintronic regions is plotted in blue. At the EPAS1 32.7-kb region, in green, is
plotted the Tibetan–Han divergence. The black arrow points to the number ofnucleotide differences between the Denisovan and the most common Tibetanhaplotype (0.0038). This value is significantly lower than what we observebetween modern human–Denisovan (red curve, P 5 0.0028).
RESEARCH LETTER
Macmillan Publishers Limited. All rights reserved©2014
Extended Data Figure 9 | Null distributions of D for an assumed Tibet–Handivergence of 3,000 years. Each histogram corresponds to the D valuesobtained under null models without gene flow, and the red vertical barcorresponds to the D values observed in the real data. The observed D values are
significant (P , 0.001) even when we assume Tibet–Han divergence of 5,000or 10,000 years (see Methods and Supplementary Tables 8–10) (modelabbreviations are given in the Supplementary Information; section onD statistics under models of no gene flow).
LETTER RESEARCH
Macmillan Publishers Limited. All rights reserved©2014
Extended Data Figure 10 | S* statistics and PCA plot. a, A measure ofintrogression, S*, from ref. 23. Distributions are for 1,000 simulations under thefour demographic models described in the Supplementary Information; sectionon D statistics under models of no gene flow. S* for the Tibetan individualsis shown as a vertical grey line. For all models, the empirical P values are 0.035,0.028, 0.019 and 0.017, respectively, for each model (top to bottom). b, Plots thefirst and second principal components using all the CHS (100 individuals)
and the CHB (97 individuals) from the 1000 Genomes and the 77 Tibetanindividuals from ref. 45 (see Methods). The black circle and the black trianglerepresent the single CHB and the CHS individuals carrying the five-SNPTibetan–Denisovan-haplotype (Extended Data Fig. 3). All SNPs in theintersection between the 1000 Genomes populations and the 77 Tibetanindividuals from chromosome 2 were used for this analysis.
RESEARCH LETTER
Macmillan Publishers Limited. All rights reserved©2014
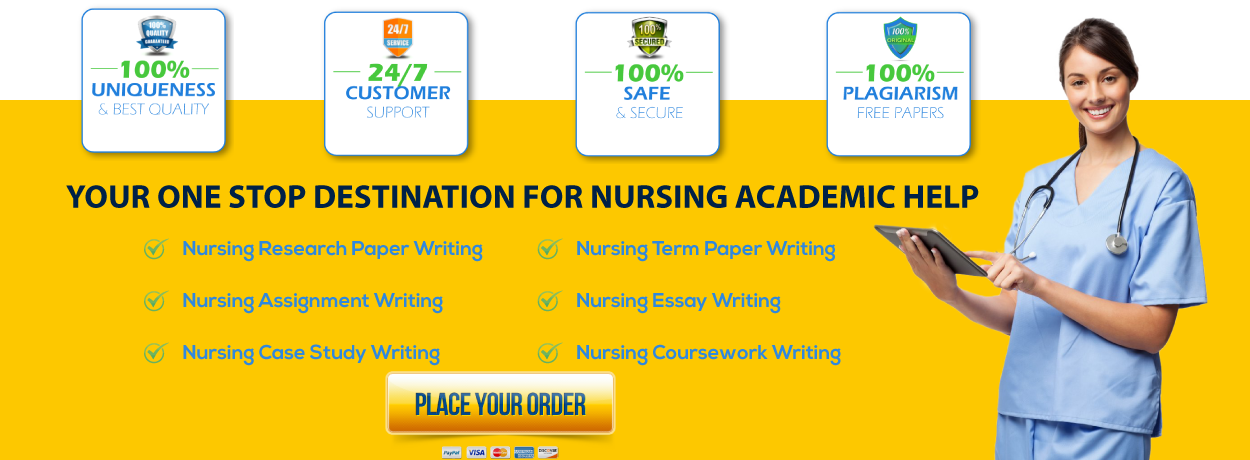